In this study, we explore the presence of biases in language models and summarization models used in legal contexts. We examine these biases through the lens of gender, race, crime against women, countries, and religious terms. Our research aims to shed light on these sensitive issues and provide insights for developers and researchers to create fairer and more neutral AI systems in the legal domain.
To tackle this complex topic, we employ various methods, including algorithmic fairness perspectives, gender bias analysis, and debiasing techniques. We evaluate our models using a variety of metrics and analyze their performance across different datasets. Our findings reveal that language models and summarization models can perpetuate biases if not properly addressed, which could lead to unfair outcomes in legal proceedings.
To address these issues, we propose several strategies for improving the fairness and neutrality of AI systems in the legal domain. These include using gender-balanced datasets, implementing debiasing techniques, and developing domain-specific abstractive models that can generate summaries that accurately reflect the key arguments, reasoning, and results of original judgments.
Our study demonstrates the importance of investigating biases in AI systems, particularly in legal contexts where fairness and impartiality are paramount. By uncovering these biases, we can work towards creating more equitable and just legal systems that serve everyone equally.
Computation and Language, Computer Science
Bias in Language Models: Analysis of Gender and Racial Stereotypes in Summaries
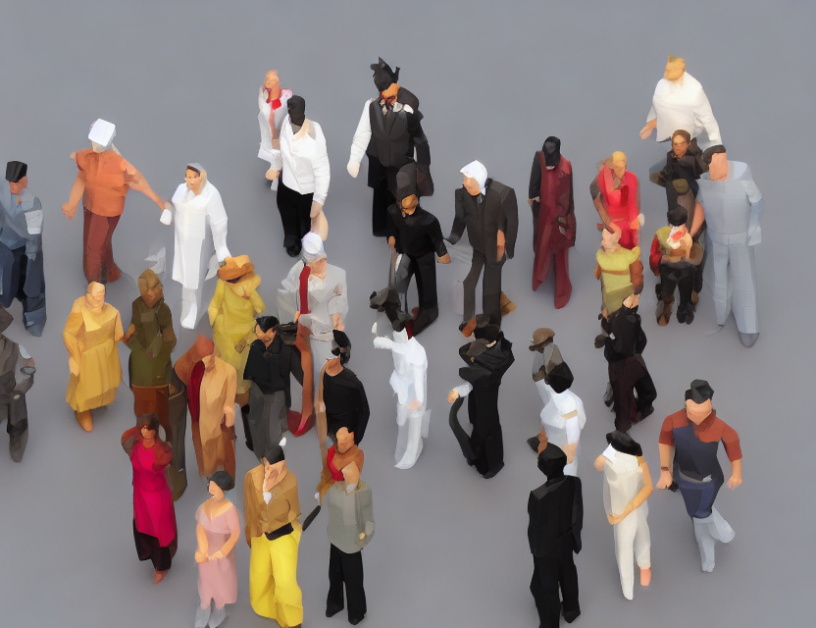