In the context of dense BeV, the anchor threshold refers to a crucial parameter that affects the performance of the attention mechanism. It determines the number of points considered active during the second pass of attention, which has a significant impact on the accuracy and efficiency of the approach.
To illustrate this concept, imagine you’re organizing a large party and want to ensure your guests are comfortable and well-entertained. You could do this by assigning specific roles to certain individuals based on their skills and interests, much like how the anchor threshold assigns importance to particular points in the BeV map. However, if too many people are assigned roles, you may end up with a chaotic and disorganized event. Conversely, if too few people are assigned roles, some guests may feel left out or uninvolved.
The ideal anchor threshold strikes a balance between these two extremes, allowing the attention mechanism to focus on the most relevant points while avoiding unnecessary complexity. This is particularly important in dense BeV, as it enables the approach to capture essential details without overwhelming the model with excessive data.
To find this optimal anchor threshold, the authors of the article vary its value and observe how it affects the distribution of activation scores for the points. They discover that a higher threshold results in fewer active points, which may lead to a loss of important information, while a lower threshold can introduce unnecessary complexity and reduce the memory efficiency of the approach.
In summary, the anchor threshold is a critical parameter in dense BeV that determines the number of points considered active during the second pass of attention. Finding the optimal value for this parameter balances the accuracy and efficiency of the approach, ensuring the best possible results without oversimplifying or overcomplicating the process.
Computer Science, Computer Vision and Pattern Recognition
Comparative Study of BeV Segmentation Methods: Reproducing and Extending Existing Work
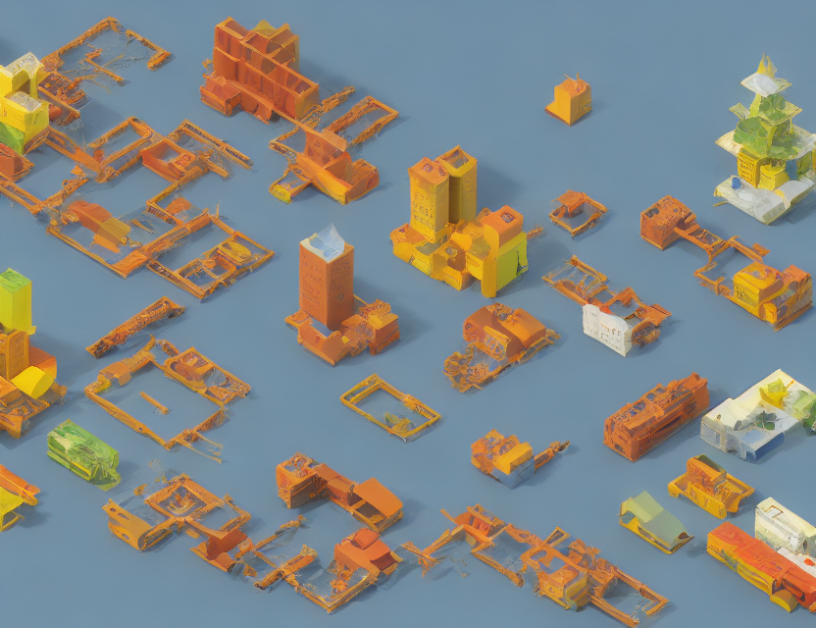