In this article, we explore the relationship between information theory and neuroscience, specifically how mathematical tools can help us understand the complex interactions between neurons in the brain. We begin by discussing the challenges of quantifying the synergy or redundancy between different parts of a system, which is crucial for understanding how information is processed in the brain.
To address this challenge, we turn to algebraic statistics, which provides a framework for analyzing complex systems. We demonstrate how this approach can be used to decompose information into its constituent parts, allowing us to understand the relative magnitudes of information and how they are related to each other.
One key insight from our work is that the landscape of mutual information can provide valuable insights into the behavior of complex systems. By analyzing the properties of this landscape, we can gain a better understanding of how information is processed in the brain and how different parts of the system interact with each other.
We also explore the homological nature of information theory, which provides a way to analyze the structure of the information landscape. This approach allows us to see how different features of the landscape are related to each other, providing a more complete understanding of the complex interactions at play.
Throughout our work, we provide numerous examples and demonstrations to illustrate the power of these methods. These include applications in neuroscience, where we show how our approach can be used to analyze the activity of neurons in the brain, as well as other fields such as computer science and engineering.
Overall, our article demonstrates the potential of algebraic statistics and information theory for understanding complex systems, including those found in the brain. By providing a framework for analyzing these systems, we hope to inspire new research and insights into the nature of information and its role in the world around us.
Computer Science, Information Theory
Decomposition of Unique Information in Multi-Source Data
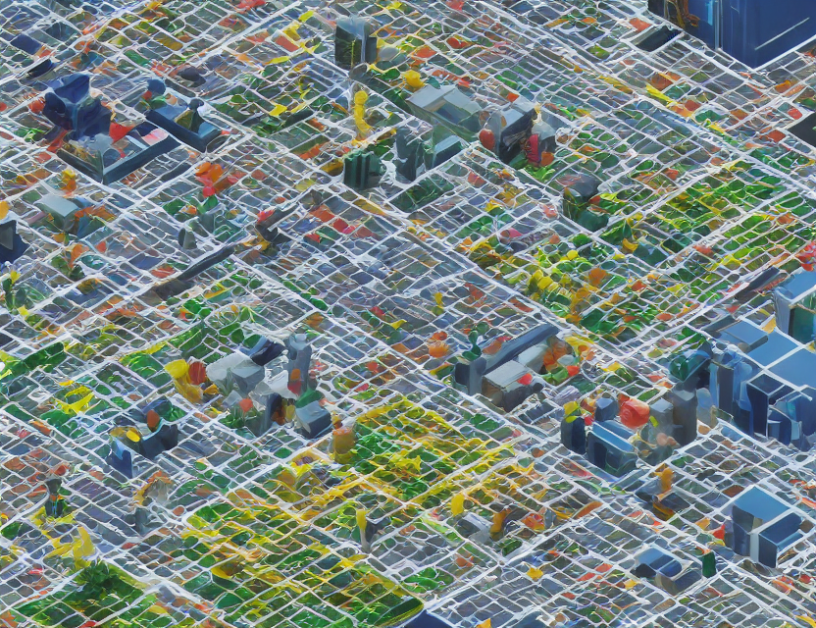