In this study, researchers aimed to develop a movement analysis system using graph convolutional neural networks (GCNNs) to detect abnormalities in neurological and musculoskeletal disorders. The system uses a combination of sensors to track the patient’s movements, such as accelerometers, gyroscopes, and magnetometers, which are then fed into a GCNN for analysis.
The GCNN is trained on a large dataset of movements from healthy individuals to learn patterns and anomalies in movement. Once the system is trained, it can be used to analyze new movements and detect potential abnormalities. The attention block in the GCNN helps focus on specific parts of the movement data that are relevant for analysis, which reduces the number of parameters required for the model.
The researchers tested their system on two datasets: one for gait analysis and another for hand function analysis. They found that their system was able to accurately detect abnormalities in both datasets with high precision.
In summary, this study demonstrates the potential of using graph convolutional neural networks (GCNNs) for movement analysis in neurological and musculoskeletal disorders. By combining multiple sensors to track patient movements and training a GCNN on a large dataset, the system can detect abnormalities with high accuracy. This technology could be used in clinics to help diagnose and monitor neurological and musculoskeletal disorders more effectively.
GCNNs are similar to traditional neural networks but have a more sophisticated architecture that allows them to learn from graph-structured data, such as movements. The attention block helps the model focus on specific parts of the movement data that are relevant for analysis, which reduces the number of parameters required for the model.
The study used two datasets: one for gait analysis and another for hand function analysis. Gait analysis involves analyzing how a person walks, while hand function analysis involves evaluating the movements of the hands. The researchers found that their system was able to accurately detect abnormalities in both datasets with high precision.
In conclusion, this study demonstrates the potential of using GCNNs for movement analysis in neurological and musculoskeletal disorders. By combining multiple sensors to track patient movements and training a GCNN on a large dataset, the system can detect abnormalities with high accuracy. This technology could be used in clinics to help diagnose and monitor neurological and musculoskeletal disorders more effectively.
Computer Science, Computer Vision and Pattern Recognition
Deep Learning for Rehabilitation: A Survey of Attention Mechanisms and Their Applications
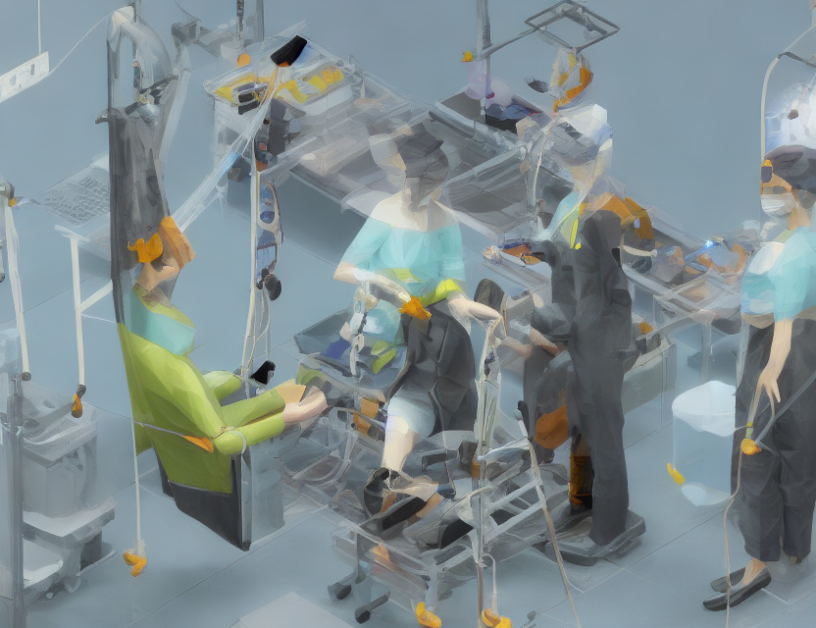