In this article, we investigate how our proposed architecture for semantic segmentation performs on two benchmark datasets: ScanNet and DynaFill. We analyze the intermediate segmentations produced by each of the three up blocks in our final architecture using a pyramid pooling module, which helps to improve the accuracy of segmentation.
Our findings show that the segmentation accuracy improves with higher feature dimensions, especially on DynaFill dataset, where the number of semantic classes is smaller and the variability is lower. However, there are still some regions in ScanNet dataset where the network struggles to produce accurate segmentations, particularly on unseen real data.
To help understand these concepts, imagine a pyramid as a tool for organizing information. The pyramid pooling module acts like a filter, extracting only the most important features from each level of the pyramid and combining them to produce more accurate segmentations. Just as a building requires a solid foundation to stand strong, our network needs these intermediate segmentations to ensure sharp and coherent boundaries in the final output.
While our architecture does not produce perfect segmentations, it is able to accurately reconstruct clean object borders and plausible semantics, leading to sharp edges and coherent textures in the resulting image and depth outputs. Think of this as a puzzle where each piece fits together perfectly to create a clear picture.
In summary, our proposed architecture improves segmentation accuracy by using pyramid pooling and analyzing intermediate segmentations on ScanNet and DynaFill datasets. While there are still areas for improvement, especially in real-world scenarios, our approach helps to demystify complex concepts in semantic segmentation and paves the way for further advancements in this field.
Computer Science, Computer Vision and Pattern Recognition
Enhancing Semantic Segmentations through Mask Design
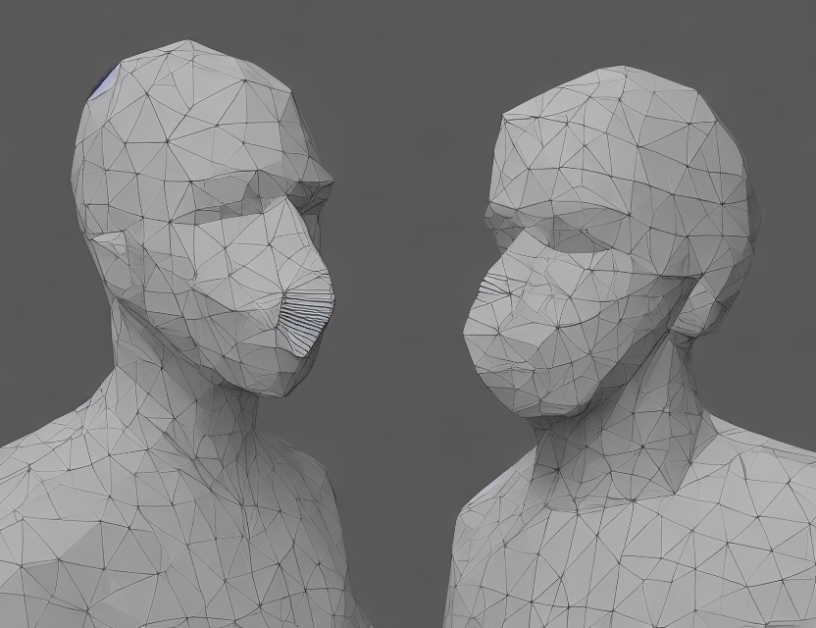