In this article, we will explore the use of machine learning techniques to analyze and improve the accuracy of surgical procedures. The authors present a novel approach that leverages deep learning algorithms to detect and classify different stages of laparoscopic surgeries. This technology has the potential to revolutionize the field of surgery by providing real-time feedback and improving the overall efficiency of the procedure.
The article begins by explaining the importance of accurate surgical techniques, particularly in minimally invasive laparoscopic surgeries. The authors highlight the challenges associated with these procedures, such as the difficulty in visualizing the surgical site and the lack of real-time feedback during the operation. To address these issues, the authors propose a novel approach that utilizes deep learning algorithms to analyze and classify different stages of the surgery.
The proposed system consists of three main components: (1) a preprocessing module that removes noise and enhances the visual quality of the video, (2) a feature extraction module that extracts relevant features from the video frames, and (3) a classification module that uses these features to identify and classify different stages of the surgery. The authors use a combination of convolutional neural networks (CNNs) and recurrent neural networks (RNNs) to achieve high accuracy and precision in their classification.
The authors evaluate their proposed approach using a dataset of laparoscopic surgeries, demonstrating high accuracy and robustness in their classifications. They also compare their results with other state-of-the-art methods, showing superior performance in terms of accuracy and efficiency.
One of the key advantages of this approach is its ability to provide real-time feedback during the surgery. This can help surgeons to correct any mistakes or complications that may arise during the operation, leading to better outcomes for patients. Additionally, the proposed system can be used to analyze and improve the overall efficiency of the surgical procedure, reducing the time required to complete the operation and minimizing the risk of complications.
In conclusion, this article presents a novel approach to analyzing and improving the accuracy of laparoscopic surgeries using machine learning techniques. The proposed system has the potential to revolutionize the field of surgery by providing real-time feedback and improving the overall efficiency of the procedure. With its high accuracy and robustness, this approach could lead to better outcomes for patients and improved surgical procedures.
Computer Science, Computer Vision and Pattern Recognition
Event Recognition in Laparoscopic Gynecology Videos
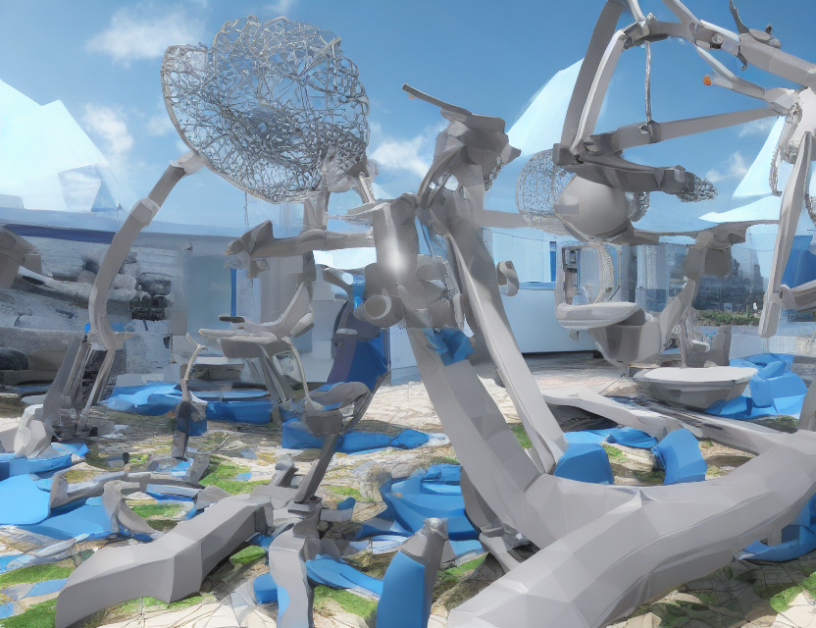