Scientific research relies heavily on data, but collecting and sharing that data can be a challenge. Currently, there are many obstacles hindering the effective curation and sharing of scientific data, including limited incentives for researchers to share quality data, difficulties in maintaining long-term data repositories, and a lack of standardized methods for evaluating the importance of various datasets. These issues make it challenging for researchers to effectively curate and share their data, which can slow down the progress of scientific research.
To overcome these challenges, the scientific community must work together to establish a fair and sustainable system for sharing data. This includes developing incentives that encourage researchers to share their data, creating long-term data repositories, and implementing standardized methods for evaluating the importance of various datasets. By doing so, we can ensure that high-quality scientific data is readily available for future generations of researchers to build upon, ultimately leading to faster advancements in our understanding of the world around us.
In simple terms, scientific data curation and sharing are crucial for the progress of scientific research. Without a fair and sustainable system in place, it becomes difficult for researchers to share their data effectively, which can slow down the pace of discovery and hinder our ability to make new breakthroughs. By working together, we can create a better system for sharing data that will benefit future generations of scientists and help us make new discoveries more quickly.
Computer Science, Networking and Internet Architecture
Expanding Scientific Data to Enhance Machine Learning Models
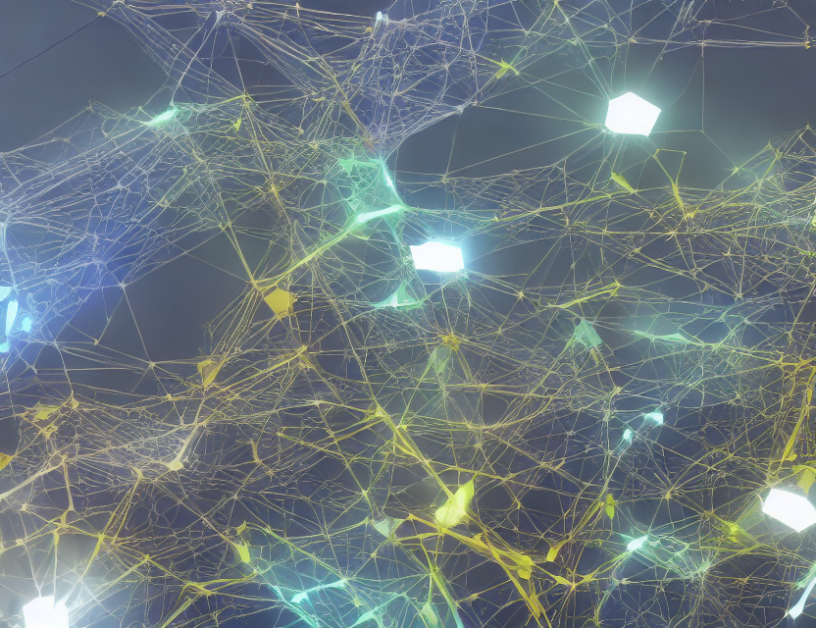