In this article, we explore the concept of generative models in visualizing abstract social processes. Generative models are AI-powered algorithms that create new images based on patterns learned from existing data. In the context of social sciences, these models can be used to visualize complex phenomena like poverty or inequality. The author discusses two taxonomies of comparative designs presented by Gleicher et al., which categorize methods into juxtaposition, superposition, and explicit encoding of relationships. These categories allow researchers to choose the most appropriate approach for their study.
To create visual representations using generative models, the author explains that semantic vectors are first determined based on a dataset of images. These vectors represent the underlying meaning or theme of each image. Once the semantic vectors have been established, the images are edited by moving in the direction of the vector from a given position or away from it in the latent space. Sampling randomly from a normal distribution with a "truncation trick" allows researchers to select latent codes with a psi value of 0.5.
The article highlights the importance of understanding generative models and their applications in social sciences. The author demystifies complex concepts by using everyday language and engaging metaphors, such as comparing the process of creating visual representations with cooking a meal. By following this approach, readers can gain a deeper comprehension of the article without feeling overwhelmed by technical jargon.
In summary, generative models provide a powerful tool for visualizing abstract social processes like poverty or inequality. By understanding how these models work and choosing the appropriate approach based on the research question, researchers can create visual representations that help them better understand complex phenomena in society.
Computer Science, Computer Vision and Pattern Recognition
Finding Semantic Vectors for Generative Image Synthesis: A Guide to Visualizing Abstract Social Processes
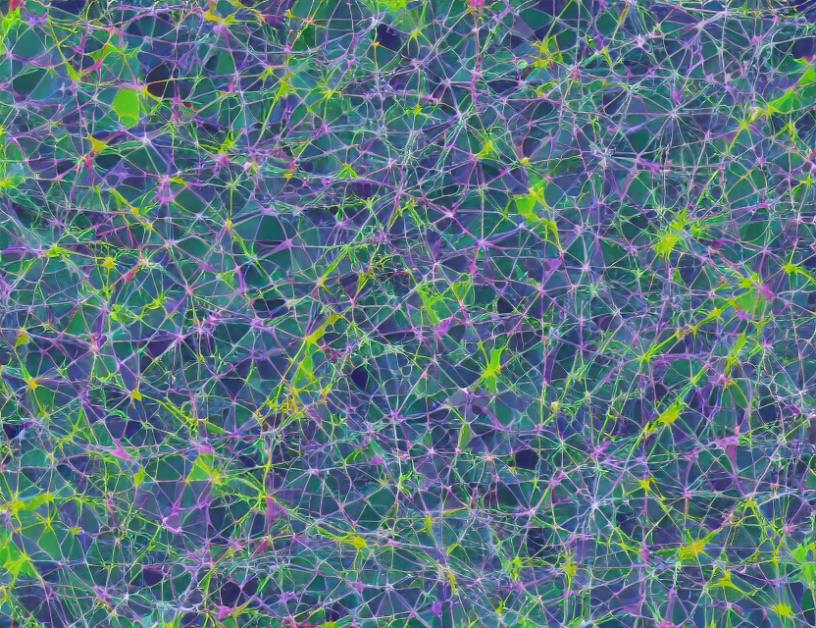