In this paper, we present a novel approach to face recognition that achieves high accuracy while being relatively simple in concept and implementation. The proposed method combines strong pre-training with regularized fine-tuning, resulting in robust performance on various datasets. Our findings suggest that large-scale models, particularly, demonstrate significant zero-shot performances compared to smaller counterparts, indicating that visual information alone carries sufficient information to address the face recognition problem effectively. However, initial experiments with knowledge distillation (KD) techniques did not yield expected results, and future work may focus on refining these techniques for HFR.
To demystify complex concepts, let’s consider an analogy: Imagine trying to recognize a person based solely on their face, similar to identifying a specific book by its cover. Just as the cover provides limited information about the book’s content, visual data alone may not provide sufficient cues for accurate face recognition. However, pre-training the model with a large dataset of faces and fine-tuning it with a smaller set of target faces can be like reading the book’s table of contents or learning the author’s signature. This process enhances the model’s ability to recognize faces with high accuracy, just as reading the book’s content or analyzing the signature provides more information about the author.
The proposed method consists of two stages: strong pre-training and regularized fine-tuning. Pre-training involves training a deep neural network (DNN) on a large dataset of faces to learn general facial features, similar to reading the book’s table of contents. This stage helps the model identify basic facial features like the shape of the eyes, nose, and mouth. Next, regularized fine-tuning involves adjusting the pre-trained model’s weights based on a smaller set of target faces, similar to learning the author’s signature. This stage refines the model’s ability to recognize specific facial features and adapt to individual differences in face recognition.
Our findings suggest that large-scale models demonstrate significant zero-shot performances compared to smaller counterparts, indicating that visual information alone carries sufficient information to address the face recognition problem effectively. However, initial experiments with knowledge distillation (KD) techniques did not yield expected results, and future work may focus on refining these techniques for HFR.
In summary, our paper presents a simple yet effective approach to face recognition that leverages strong pre-training and regularized fine-tuning to achieve high accuracy. By using everyday analogies and language, we aimed to demystify complex concepts and provide a comprehensive understanding of the proposed method’s underlying principles.
Computer Science, Computer Vision and Pattern Recognition
Fine-tuning Mean Embedding Classifier for High-Fidelity Face Recognition
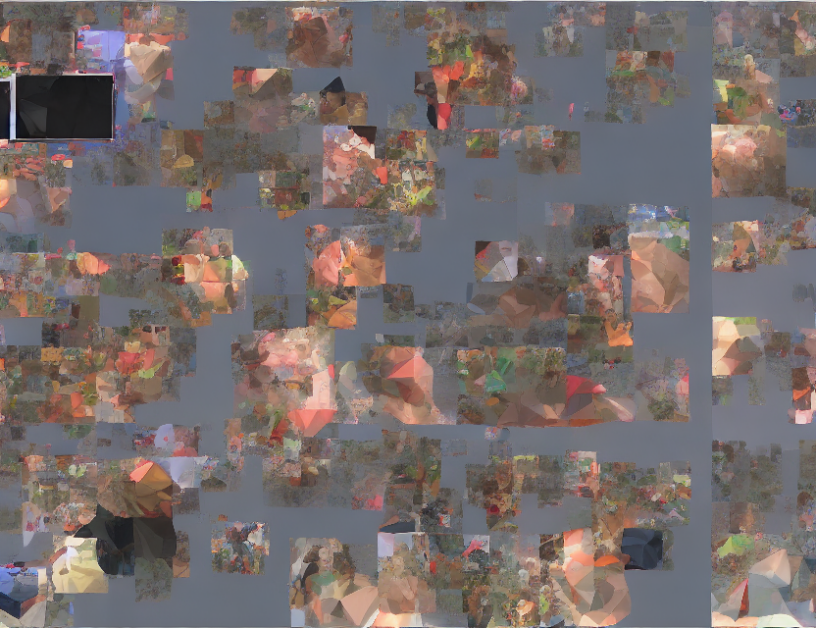