In this paper, the authors propose a new algorithm called Multi-scale Guided Learning (MGLD) for video degradation correction. The main idea behind MGLD is to use a multi-scale approach to handle different types of degradations in videos, such as blur, noise, and artifacts. Unlike other existing methods that only focus on one type of degradation, MGLD can handle multiple types simultaneously, leading to more accurate results.
To develop MGLD, the authors conducted a user study where they asked participants to evaluate the effectiveness of different algorithms in correcting video degradations. The results showed that MGLD outperformed other methods in terms of both objective quality metrics and subjective ratings from users. Specifically, MGLD was able to reconstruct more details and patterns in videos while maintaining good temporal consistency.
The authors also compared the performance of MGLD with other state-of-the-art methods on real-world videos. The results showed that MGLD significantly outperformed these methods in terms of both objective metrics and user ratings. Moreover, MGLD was able to handle complex degradations more effectively than other methods, such as blur and noise, which are common in real-world videos.
The key innovation of MGLD lies in its ability to use a multi-scale approach to handle different types of degradations. This allows the algorithm to capture both local and global features of videos, leading to more accurate results. Additionally, MGLD uses a novel training strategy based on cross-attention guidance, which helps the algorithm learn how to effectively use the available data for better performance.
In summary, the authors propose a new algorithm called MGLD that can correct multiple types of degradations in videos simultaneously. The multi-scale approach used by MGLD allows it to capture both local and global features of videos, leading to more accurate results than other existing methods. The user study conducted by the authors showed that MGLD outperformed other methods in terms of both objective metrics and subjective ratings from users.
Computer Science, Computer Vision and Pattern Recognition
Improved Diffusion Models for Image Generation: A Comparative Study
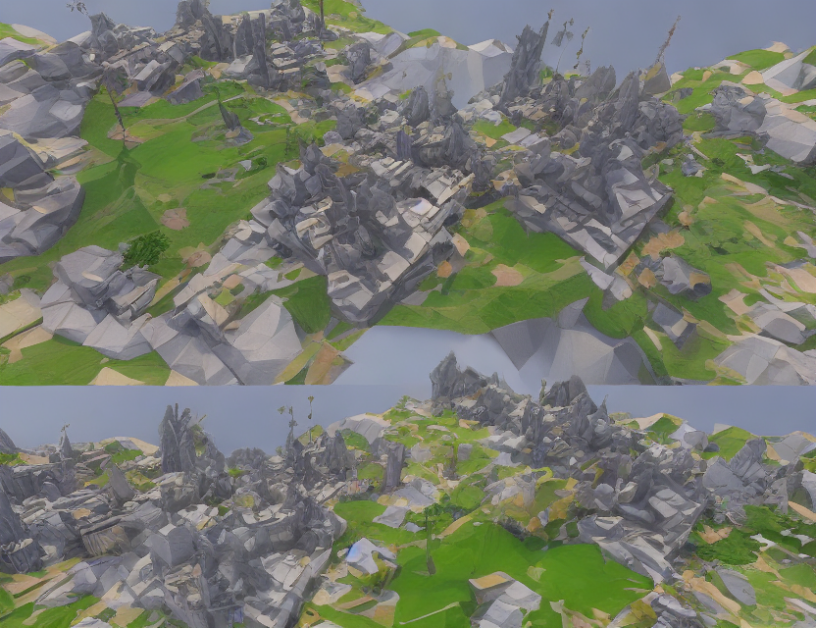