One advantage of our modular approach is that we can define different modules better adapted to certain tasks, such as understanding higher-level semantics. For example, when asked which option best describes the overarching narrative of the video, a human would construct a mental narrative and then match it with the available options. Our module can decompose a query into steps, translate them into function calls, and execute them to procedurally obtain an answer.
In conclusion, our modular approach to long video summarization combines the use of object detectors, retrieval methods, captioning, and image QA to solve complex questions zero-shot. By breaking down a query into smaller steps and using pre-trained models to execute each step, we can produce qualitatively accurate summaries that capture the essence of the video without oversimplifying.
Computer Science, Computer Vision and Pattern Recognition
Improving Multimodal Question Answering with In-Context Learning
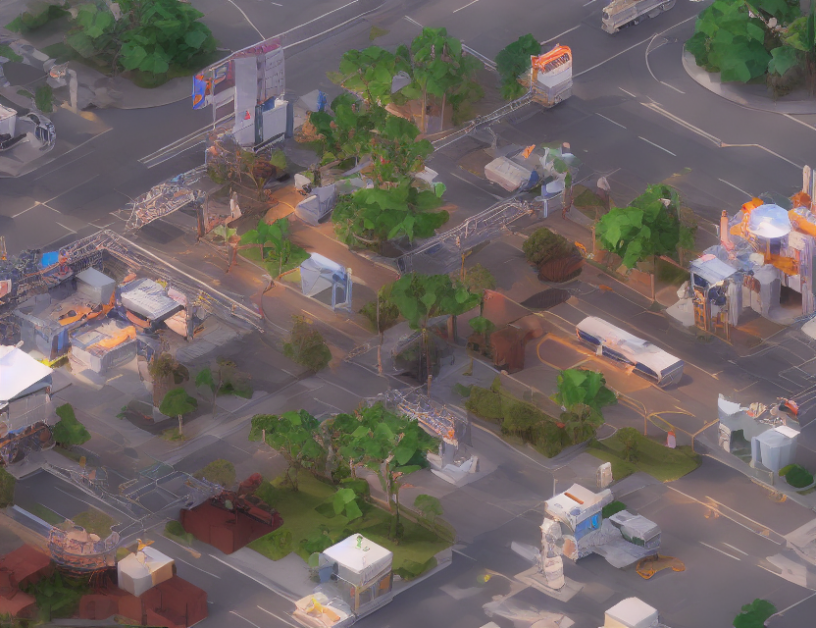