In this article, researchers propose a new method called Lightweight Attentional Feature Fusion (LAFF) for text-to-video retrieval. The goal is to improve the efficiency and accuracy of video retrieval systems by leveraging attentional mechanisms that focus on relevant parts of the input text and video.
Imagine you’re browsing a vast library of videos and need to find the perfect match for a given text query. The task can be challenging, as each video is a complex mixture of visual and audio content. To address this problem, researchers propose LAFF, which combines two key elements: attentional feature fusion and lightweight feature representation.
Attentional feature fusion allows the system to focus on the most relevant parts of the input text and video by computing attention weights based on their similarity. This process helps to reduce the dimensionality of the input data and improve the efficiency of the subsequent processing steps. Lightweight feature representation, on the other hand, reduces the number of parameters in the model, making it easier to train and more energy-efficient to deploy.
The proposed LAFF method outperforms existing state-of-the-art methods in text-to-video retrieval tasks, achieving better accuracy while reducing computational costs. The authors evaluate their approach on several benchmark datasets and show that it can efficiently retrieve relevant videos for a given query while ignoring irrelevant ones.
In summary, LAFF is a powerful tool for improving the efficiency and accuracy of text-to-video retrieval systems. By combining attentional feature fusion with lightweight feature representation, researchers have developed a method that can quickly and accurately retrieve relevant videos from vast datasets. This innovative approach has significant implications for various applications, such as video recommendation systems, search engines, and virtual reality environments.
Computer Science, Computer Vision and Pattern Recognition
Improving Video Retrieval via Linking Characters to Visual Representations
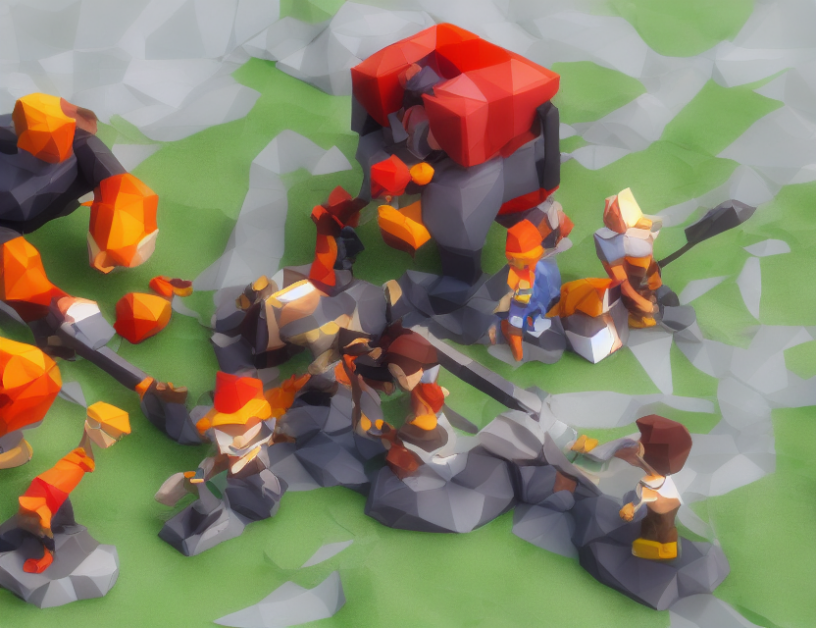