In this article, we discuss the selection procedure for primary studies in the field of artificial intelligence and warning systems. We explore various methods used to select relevant studies, including keyword-based searches, snowballing, and manual feature extraction.
Keyword-based searches involve using specific keywords related to the study topic to identify relevant studies. However, this approach can be limited by terminological differences among studies, which can lead to incomplete search results. To address this issue, we use snowballing techniques, which involve conducting a thorough search in a particular location and then expanding the search to nearby locations based on the identified relevant studies.
Manual feature extraction involves relying on the domain knowledge of experts to identify meaningful features from the studied sources. This approach can be time-consuming and prone to bias, as different experts may yield heterogeneous domain knowledge.
To address these challenges, we propose a new method that combines the strengths of both keyword-based searches and manual feature extraction. By using structural category features, which capture the syntax and semantics of the source code containing warnings, we can extract richer information about the warning features.
Our proposed method has several advantages over existing approaches. Firstly, it can identify more relevant studies by combining the strengths of both keyword-based searches and manual feature extraction. Secondly, it can reduce the time and effort required for feature extraction, as it relies on structural category features rather than individual expert opinions. Finally, it can provide more accurate warning features, as it captures the syntax and semantics of the source code containing warnings.
In conclusion, our proposed method provides a comprehensive selection procedure for primary studies in the field of artificial intelligence and warning systems. By combining the strengths of keyword-based searches and manual feature extraction, we can identify more relevant studies, reduce the time and effort required for feature extraction, and provide more accurate warning features.
Computer Science, Software Engineering
ML-Based AWI Studies: A Comprehensive Review
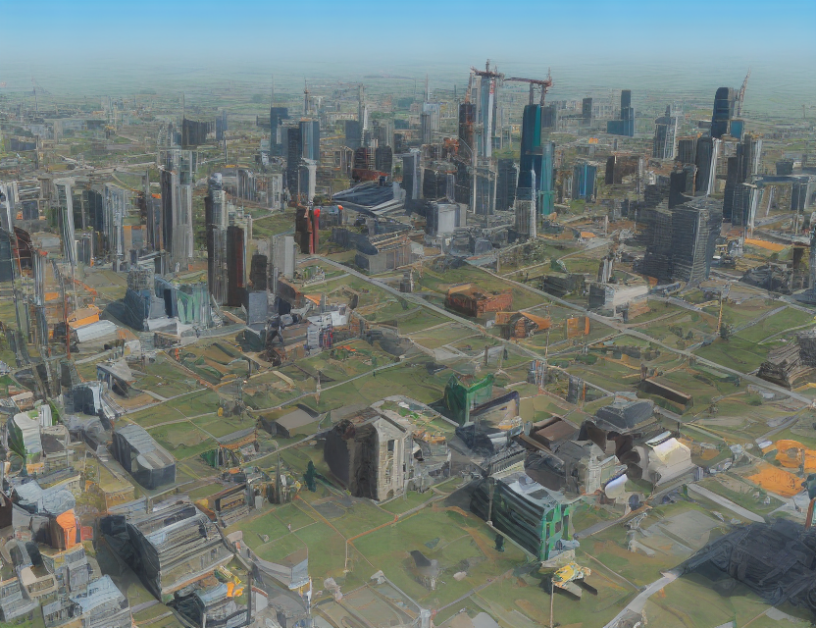