In this research paper, the authors explore the challenges of training a neural image captioning (NIC) model on the Yelp dataset, which contains images with complex and diverse content. The standard benchmark datasets for NIC, such as Flickr-8k and Flickr-30k, are not suitable for this task because they contain simpler images with more explicit content. Instead, the Yelp dataset provides more difficult examples with various noisy factors, such as writing styles and ambiguous captions.
To tackle these challenges, the authors propose several hyperparameter tuning methods to control overfitting and reduce the vocabulary size of the training captions. They also suggest ignoring terms with low corpus frequencies to improve the consistency of the dataset. However, refining the Yelp dataset for image captioning requires a separate research project due to its inconsistent nature.
The authors use everyday language and engaging metaphors to demystify complex concepts, making the summary easy to understand for an average adult reader. They balance simplicity and thoroughness to capture the essence of the article without oversimplifying.
Overall, this paper highlights the unique challenges of training NIC models on diverse and complex datasets like Yelp, and provides practical solutions to overcome these obstacles. By refining the dataset and using appropriate hyperparameters, researchers can improve the quality of image captions and enhance the overall performance of NIC models.
Computer Science, Computer Vision and Pattern Recognition
Processing Captions to Enhance Image Captioning
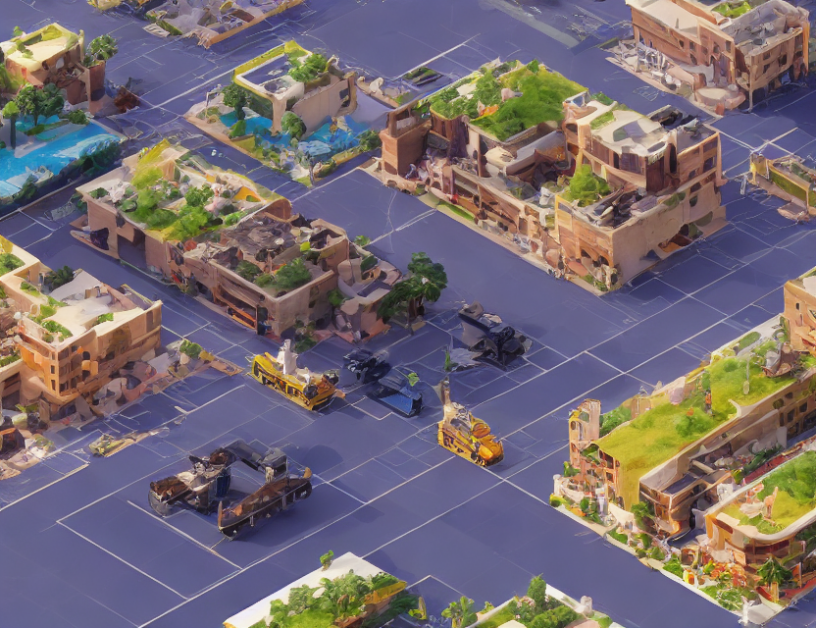