This paper proposes a novel approach to detecting stealthy cyber attacks in constrained cyber-physical systems. The proposed method leverages moving target and watermarking ideas, as well as dimensionality reduction, optimal reconstruction, and time-varying encoding mechanisms to identify hidden threats. The system can detect both stealthy replay and covert attacks, providing enhanced security for critical infrastructure.
The authors explain that traditional detection methods are insufficient in today’s increasingly complex cyber landscape, where stealthy attacks are becoming more common. These techniques rely on detecting anomalies in system behavior, but such attacks can be disguised to blend in with normal activity. The proposed method addresses this limitation by using a proactive approach that anticipates and adapts to evolving attack patterns.
The authors present two case studies demonstrating the effectiveness of their approach. In Case A, the system detects a stealthy replay attack with 100% accuracy, while in Case B, it accurately identifies a covert attack hidden within normal system activity. The results show that the proposed method can significantly improve security in constrained cyber-physical systems.
The authors also discuss the limitations of their approach and suggest future research directions. They emphasize the importance of continuously updating and refining the detection mechanisms to stay ahead of evolving attack techniques.
In summary, this paper presents a cutting-edge solution for detecting stealthy cyber attacks in constrained systems. By leveraging advanced analytics and machine learning techniques, the proposed method offers enhanced security features that can help protect critical infrastructure from emerging threats.
Computer Science, Systems and Control
Recovering Tracking Performance after Cyber-Physical Attacks with Integrated Safety Verification
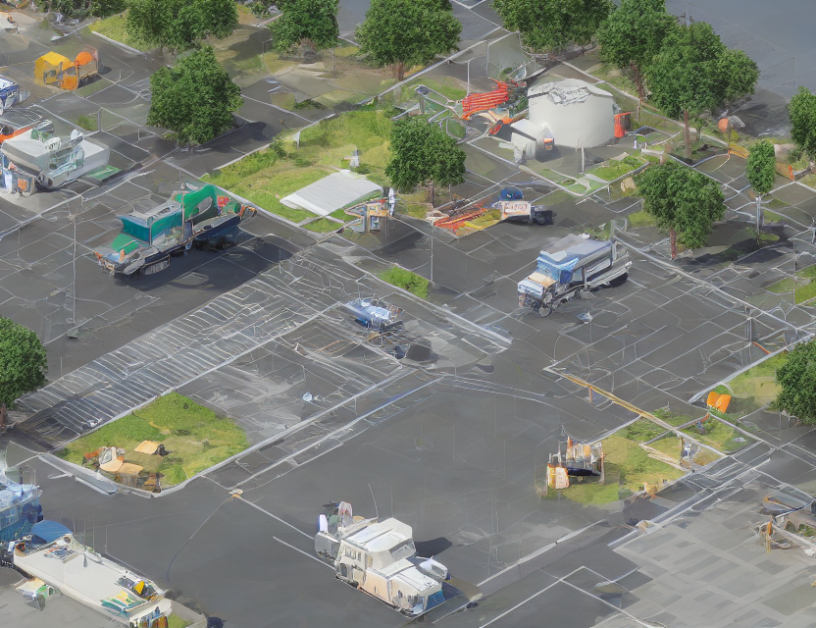