In this article, we explore the potential of recent text-to-image synthesis methods for generating high-quality images that adhere to physical constraints. We introduce the concept of "diffusion models," which work by reversing a diffusion process that adds noise to high-quality images and can generate high-quality samples from various distributions. These models have gained popularity in recent years due to their ability to generate detailed and realistic images, but they are limited by their reliance on vast datasets and text encoders for priors on scene composition and object properties.
To address this limitation, we propose a new approach that combines diffusion models with inpainting techniques described in [Lugmayr et al. 2022]. Our method allows us to take our general text-to-image diffusion models and perform inpainting using the techniques described by [Lugmayr et al. 2022]. We evaluate the results using the LPIPS metric, which measures the perceptual similarity between two images using features from deep neural networks. The results show that our method can generate high-quality images that are similar to the original image but with missing regions inpainted.
We also explore the use of monocular depth estimation models for generating 3D scenes from a single image. These models have been shown to be effective in recovering 3D planes from a single image via convolutional neural networks [Fengting Yang and Zihan Zhou. 2018]. We demonstrate that our method can generate high-quality 3D scenes by scaling autoregressive models for content-rich text-to-image generation [Jiahui Yu, et al. 2022].
In summary, this article presents a new approach to text-to-image synthesis that combines diffusion models with inpainting techniques to generate high-quality images that adhere to physical constraints. The proposed method demonstrates the potential of recent text-to-image synthesis methods for generating detailed and realistic images while addressing their limitations. Additionally, the article explores the use of monocular depth estimation models for generating 3D scenes from a single image, which has applications in various fields such as robotics and computer vision.
Computer Science, Computer Vision and Pattern Recognition
Training Latent Diffusion Models for Image Generation
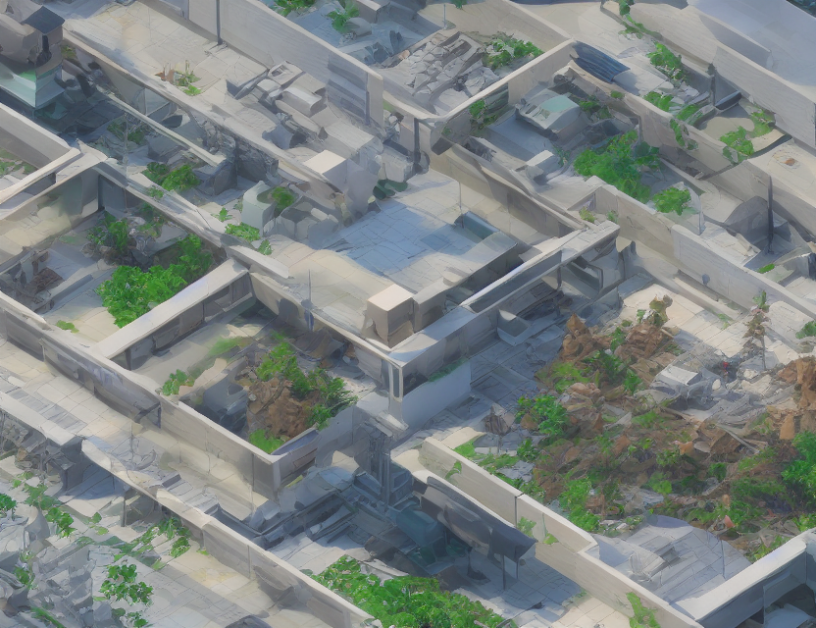