In this research paper, the authors aim to develop a novel dataset and approach for extracting explanations for correct answers in medical question answering tasks. They argue that existing datasets, such as SQuAD, are too simplistic and do not capture the complexity of real-world medical questions. To address this issue, they create a dataset called CasiMedicos, which includes longer answers with supporting explanatory arguments.
The authors evaluate their dataset using various Spanish language models and find that multilingual non-specialized models often outperform monolingual ones, even those pre-trained with large amounts of medical texts. They also show that their novel approach can be an effective technique for identifying relevant evidence-based explanations for medical questions.
The authors use the metaphor of a "journey" to explain their approach, stating that they want to help medical practitioners navigate through a vast amount of unstructured text to find the most relevant and timely information. They also use the term "evidence-based medicine" to describe the goal of using scientific information extracted from systematic reviews to guide clinical decision-making.
The authors emphasize that their approach is designed to be more complex than existing datasets, with answers that can range from 32 words long to 3.2 words per answer in SQuAD. They also highlight the importance of demystifying complex concepts by using everyday language and engaging metaphors or analogies to capture the essence of their research without oversimplifying.
Overall, the authors aim to provide a novel approach for extracting explanations for correct answers in medical question answering tasks, focusing on the complexity of real-world medical questions and the importance of using evidence-based medicine to guide clinical decision-making.
Computation and Language, Computer Science
Uncovering the Truth: How Misconceptions in Medical Texts Can Lead to Deception
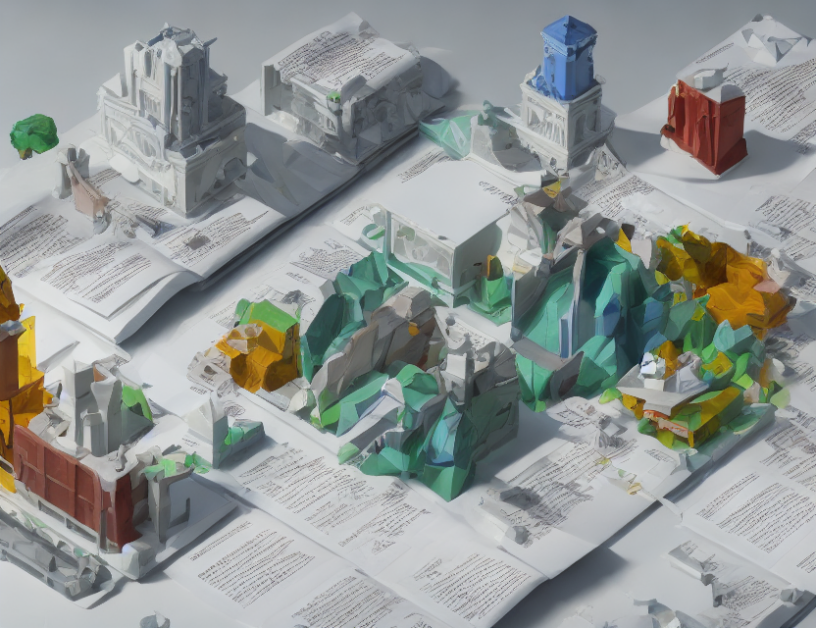