Scene understanding is a fundamental aspect of human intelligence, allowing us to interpret and navigate complex environments effortlessly. However, developing AI systems that can match human capabilities in this regard remains an elusive goal. To address this challenge, researchers have turned to unsupervised object-centric representation learning, which seeks to represent a scene as a composition of distinct objects using only visual information.
In this article, we delve into the world of unsupervised object-centric representation learning, exploring its potential to demystify scene understanding. We begin by introducing the context, highlighting the importance of object-centric representations in real-world data and the crucial role of decoders in slot-based autoencoders.
The key insight behind unsupervised object-centric representation learning is that humans can typically achieve scene decomposition with visual cues alone, without needing any labeled data. Inspired by this observation, researchers have developed various techniques to learn object-centric representations from real-world image data. One such approach involves distilling feature correspondences, which helps the model understand how features relate to objects in the scene.
To further enhance their capabilities, researchers have also explored the use of causal self-attention layers and auto-regressive transformer decoders. These innovations enable the model to predict the feature at each position based on prior target features and slots, leading to more accurate and efficient representation learning.
The article concludes by highlighting the potential of unsupervised object-centric representation learning to revolutionize scene understanding in AI systems. By leveraging the wealth of unlabeled image data available, these techniques can help create AI systems that can interpret and navigate complex environments with ease, matching human intelligence in a wide range of applications.
In summary, this article sheds light on the power of unsupervised object-centric representation learning to transform scene understanding in AI systems. By replicating the way humans process visual information, these techniques have the potential to create more sophisticated and efficient AI agents capable of tackling complex real-world tasks with ease.
Computer Science, Computer Vision and Pattern Recognition
Unsupervised Object-Centric Learning for Real-World Videos
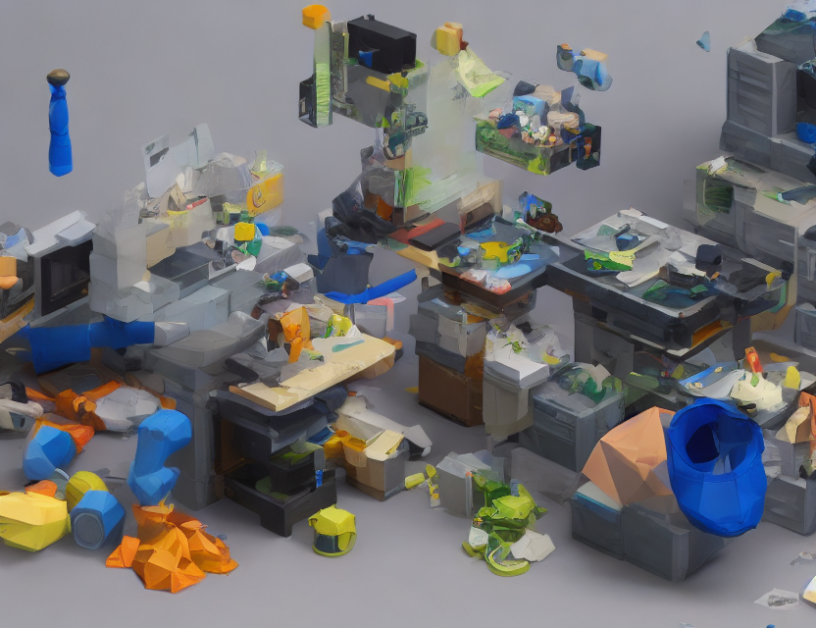