In this paper, we propose a novel approach to interpreting machine learning models based on vessel trajectory data. Our method combines several established XAI techniques, such as LIME, SHAP, saliency maps, attention mechanisms, direct trajectory visualization, and Permutation Feature Importance. By integrating these methods, we create a comprehensive framework that provides deeper and more granular insights into the decision-making process of models relying on vessel trajectory data.
To validate our approach, we conducted a survey among various user demographics, including professionals with academic backgrounds and end-users without extensive knowledge of AI and Data Science. Our findings revealed a dichotomy between these groups, with professionals favoring technical methods for interpretability and end-users preferring simpler visualizations like bar plots and visual depictions of critical parts of a vessel’s trajectory.
Our proposed method offers several advantages, including the ability to visualize trajectories and identify key model influencers, as well as the capacity to build trust with users by providing explanations for model predictions. By combining attention mechanisms, LIME, and SHAP, we create a robust interpretability matrix that produces insights rooted in the model while remaining agile enough to span diverse models.
In summary, our approach provides a multifaceted solution to the complex challenge of interpreting machine learning models based on vessel trajectory data. By demystifying complex concepts and using everyday language, we aim to empower users to comprehend the decision-making process of these models with confidence.
Artificial Intelligence, Computer Science
Unveiling Trajectory Data Insights: A Comprehensive XAI Approach
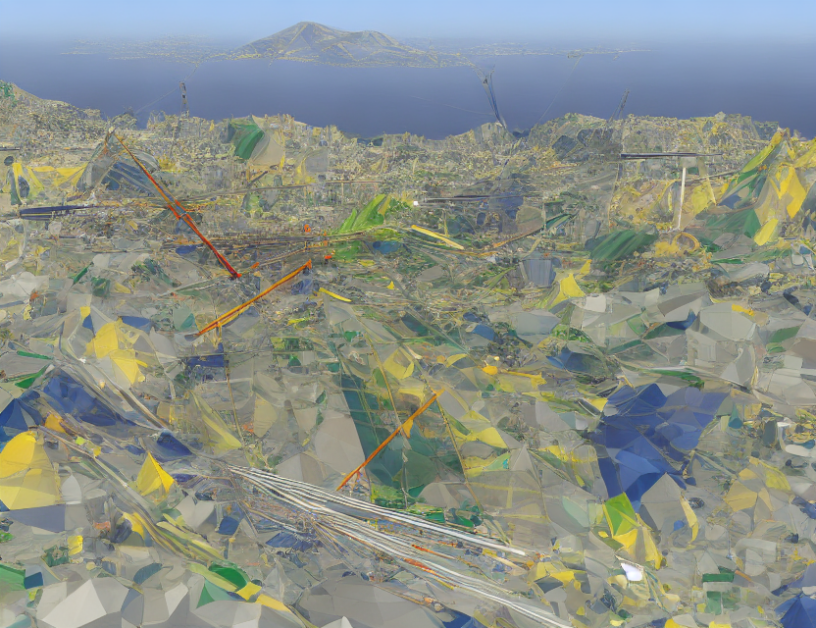