In this article, we explore the concept of audio-visual fusion and its significance in improving performance in various cognitive tasks. The authors propose a novel model called DeepAVFusion that combines both modalities at an early stage to leverage their complementary information. They demonstrate the effectiveness of this approach through experiments on several tasks, including object recognition, face recognition, and visual question answering.
The key insight behind DeepAVFusion is that audio and visual modalities contain different types of information that can enhance each other’s performance when combined early in the processing pipeline. By fusing these modalities, the model can learn to represent complex stimuli more effectively, leading to better performance across various tasks.
To understand how this works, think of it like cooking a meal. Imagine you have two separate ingredients – one for the visual portion (like vegetables) and another for the audio portion (like spices). By combining them early on, you create a more flavorful and nutritious dish that can be enjoyed by everyone. Similarly, DeepAVFusion combines the visual and audio modalities to create a more robust representation of the stimuli, leading to better performance in various tasks.
The authors also explore the impact of different design choices on the model’s performance. They find that early fusion is crucial for optimal performance, and that the number of fusion layers and aggregation tokens can significantly affect the model’s ability to represent complex stimuli effectively.
Overall, this article demonstrates the importance of audio-visual fusion in improving performance in various cognitive tasks. By leveraging the complementary information present in both modalities, DeepAVFusion shows promising results that could have real-world applications in fields like robotics, autonomous driving, and human-computer interaction.
Computer Science, Computer Vision and Pattern Recognition
Attention-Based Models Lead to Breakthroughs in Audio-Visual Scene Analysis
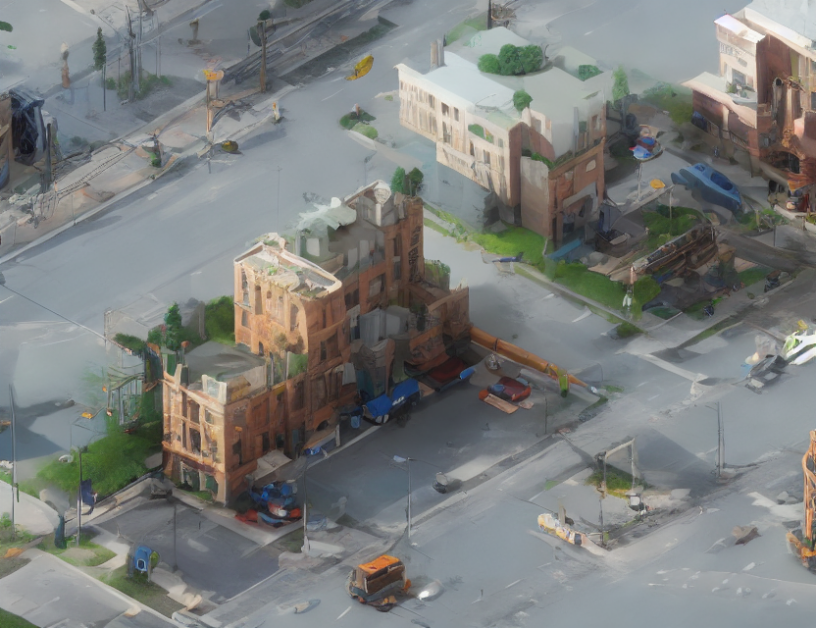