In recent years, there has been a growing interest in analyzing the sentiment of text at the perspective level, which involves determining the sentiment polarity of each perspective word expressed in a text. This review aims to provide a comprehensive overview of the current state-of-the-art methods for perspective-level text sentiment analysis, highlighting their strengths and limitations.
One of the key challenges in perspective-level sentiment analysis is handling the differences in sentiment between perspective words and their left and right contexts. To address this issue, some researchers have proposed incorporating phase information into convolutional neural networks (CNNs) using parameterized filters and threshold mechanisms. Other approaches include using semantic cognitive networks to simulate the cognitive process of human reading and better capture the relationship between context and perspective words.
However, these methods have limitations in terms of considering the different degrees of contribution of words to sentiment categorization in different perspectives. Moreover, logical rules for knowledge graph reasoning are often overlooked, which can result in inaccurate sentiment classification.
To address these challenges, attention mechanisms have been proposed in recent years. These mechanisms allow the model to focus on specific parts of the input text that are relevant to the task at hand, improving the accuracy of sentiment analysis. Various models, such as MemNet, IANs, and RAM, have introduced attention mechanisms to address the issue of treating all words equally in sentiment classification.
In conclusion, perspective-level text sentiment analysis is a rapidly evolving field with many exciting developments in the area of attention mechanisms. By better capturing the relationship between context and perspective words, these methods have shown promising results in improving the accuracy of sentiment classification. As the field continues to grow, it is likely that we will see even more innovative approaches to addressing the challenges associated with perspective-level text sentiment analysis.
Computation and Language, Computer Science
Attention-based Sentiment Analysis Uncovers Dreadful Service
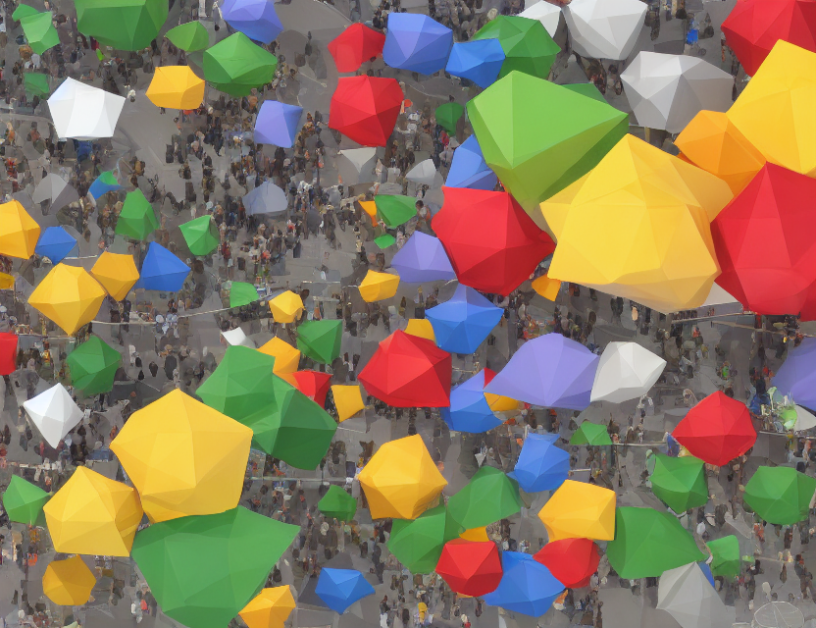