In today’s digital age, signatures are widely used for identity verification in various scenarios, including legal documents, financial transactions, and medical consents. However, with the increasing sophistication of scams, manual signature verification has become impractical. To address this challenge, researchers have developed automated signature verification systems using machine learning techniques.
Deep generative models, such as Generative Adversarial Networks (GANs), have shown promising results in signature verification tasks. These models can generate high-quality synthetic signatures that are difficult to distinguish from real signatures. However, the generated signatures may not accurately reflect the true identity of the signer, making it challenging to evaluate their authenticity.
To address this challenge, the article proposes an alternative approach for evaluating the quality of generated signatures using a data-driven verification system. The proposed system utilizes a feature-based method to extract relevant information from the signatures and compare them with the original signature. The system also employs an attack explainability mechanism to identify potential vulnerabilities in the generated signatures, providing insights into the factors that can compromise their authenticity.
The article presents a comprehensive evaluation of the proposed system using a dataset of signatures from various individuals. The results demonstrate the effectiveness of the system in accurately verifying signatures while also providing valuable insights into the quality of the generated signatures.
In summary, the article proposes a novel approach to automated signature verification using deep generative models and a data-driven verification system. The proposed system provides an effective means of evaluating the authenticity of generated signatures while also identifying potential vulnerabilities in the verification process. By leveraging cutting-edge machine learning techniques and feature extraction methods, the article demonstrates a significant improvement over traditional signature verification approaches.
Computer Science, Computer Vision and Pattern Recognition
Conditional Generative Adversarial Networks for Synthetic Data Generation in Offline Writer-Independent Signature Verification
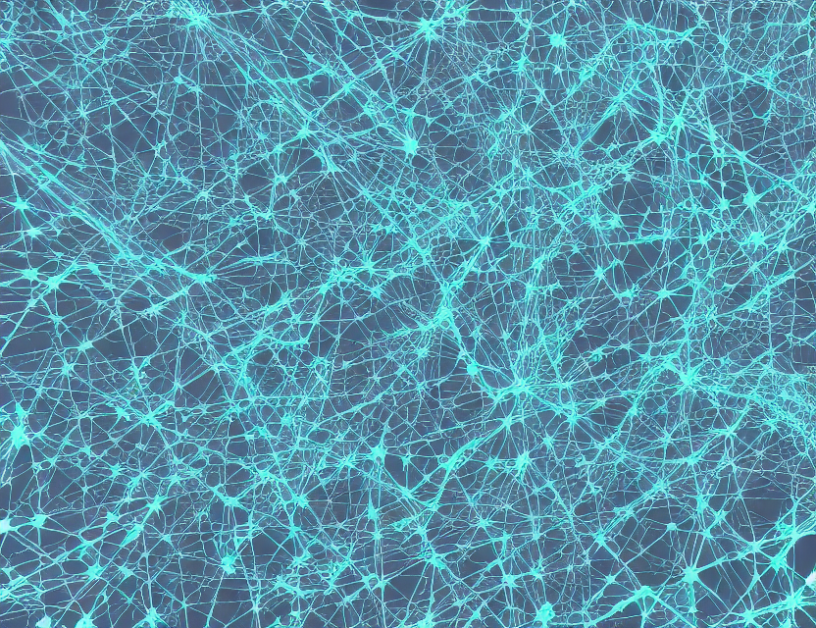