Text-to-image synthesis is a rapidly growing field that allows us to generate images based on text descriptions. Recently, diffusion models have gained popularity in this area due to their ability to capture complex structures and details. However, these models can be challenging to interpret, making it difficult to understand why they produce certain images. In this article, we aim to demystify diffusion models by exploring their inner workings and highlighting their strengths and weaknesses.
The article begins by explaining the concept of diffusion models and how they differ from other text-to-image synthesis methods. The authors then delve into the inner workings of diffusion models, discussing the various components involved in the generation process. They explain that these components include a latent diffusion model (LDM) and a decoder network, which work together to produce high-quality images.
One of the key strengths of diffusion models is their ability to capture complex structures and details. The authors provide examples of how these models can generate images with intricate patterns and textures, such as leaves or clouds. However, they also highlight a potential weakness of these models: their reliance on noise-free training data. If the training data contains noise, the models may struggle to produce accurate images.
To address this issue, the authors propose a new method called conditional control, which adds an additional term to the loss function to encourage the model to generate cleaner images. They demonstrate the effectiveness of this approach on several datasets and show that it can significantly improve the quality of the generated images.
The article also includes an ablation study that compares the performance of diffusion models with other state-of-the-art methods. The results show that diffusion models outperform these methods in terms of both structural information and detail preservation. This further reinforces the idea that diffusion models are a promising approach to text-to-image synthesis.
In conclusion, this article provides a comprehensive overview of diffusion models for text-to-image synthesis, highlighting their strengths and weaknesses. By demystifying these models, the authors provide insights into how they work and why they produce certain images. These findings can help researchers and practitioners improve their understanding of diffusion models and develop new methods that build upon them.
Computer Science, Computer Vision and Pattern Recognition
Generating Powerful Images with Diffusion Models
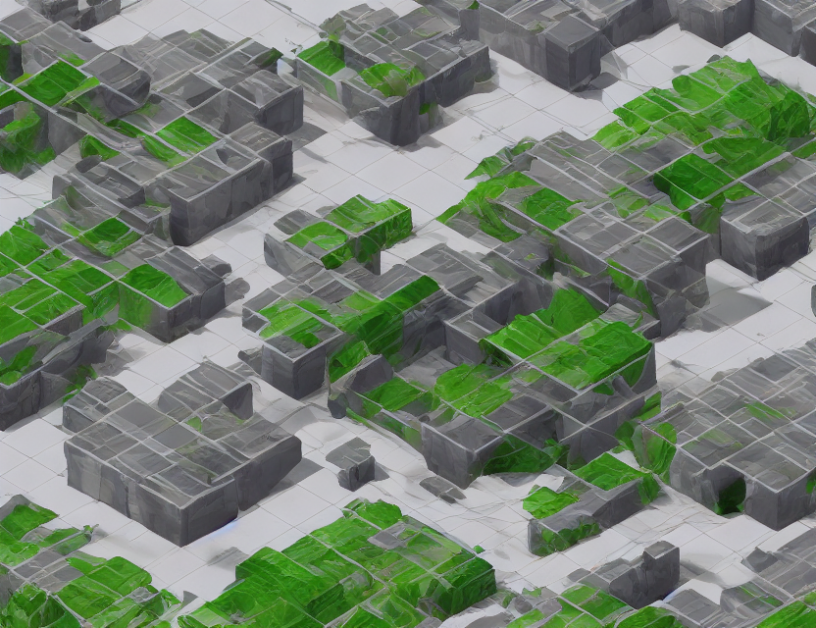