Automatic evaluation metrics for text simplification have been widely used, but their suitability has been questioned due to issues with grammar, appropriateness, relevance, and novelty. Fernando Alva-Manchego et al. (2021) conducted a study to investigate the problems with these metrics and proposed a new approach that considers the complexity and novelty of the generated text.
The study used two datasets, one for training and another for testing, which consisted of texts from various platforms such as Wikipedia articles. The authors found that the kappa values in grammar, appropriateness, relevance indicate a moderate agreement, while the kappa results for complexity and novelty indicate a fair level of agreement.
The study also showed that most questions in the datasets do not incorporate multiple sentences as their basis, and there is a notable QG dataset for educational purposes called LearningQ, which utilizes complete articles or videos as contexts. In contrast, the proposed approach utilizes explanatory answers that contain comprehensive knowledge points relevant to the question.
In conclusion, the study highlighted the limitations of existing automatic evaluation metrics for text simplification and proposed a new approach that considers the complexity and novelty of the generated text. The proposed approach is more thorough and provides a better balance between simplicity and thoroughness, making it a valuable contribution to the field.
Computation and Language, Computer Science
Improving Question Generation with Prompt-Based Fine-Tuning and Multimodal Paraphrasing
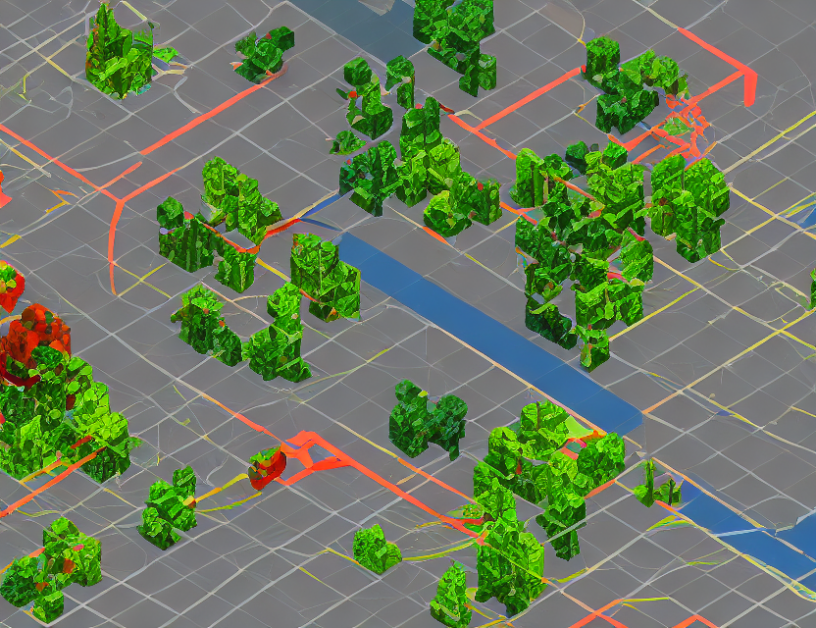