In this article, we explore the limitations of using context-independent representations in natural language processing (NLP). We examine the concept of context-independent representations, which assume that a model’s understanding of language is independent of the context in which it is used. This idea has been influential in the field of NLP, but we find that it is implausible to achieve context-independent representations for all personas.
We demonstrate this by exploring the use of contrast pairs, which drive the empirical performance of contrast consistent search (CCS). We show that probes generalize well from easy to hard examples, indicating that the model’s understanding of language is influenced by the context in which it is used. This challenges the idea of context-independent representations and highlights the importance of considering context when training NLP models.
To further investigate the limits of context-independent representations, we propose two lines of inquiry: (1) investigating the "persona capacity" of the residual stream, which could shed light on the extent to which a model’s understanding of language is influenced by the context in which it is used; and (2) exploring the causal mechanisms involved in shaping the model’s responses. By answering these questions, we hope to gain a deeper understanding of the nature of context-dependent representations in NLP and their potential applications.
In summary, our article challenges the idea of context-independence in NLP by demonstrating that models are influenced by the context in which they are used. We propose lines of inquiry to explore the limits of context-independent representations and shed light on the complex relationships between language, context, and representation. By demystifying these concepts, we aim to advance our understanding of how NLP works and develop more effective models for a range of applications.
Computer Science, Machine Learning
Large Language Models’ Reliability on Human-Generated Labels Questioned
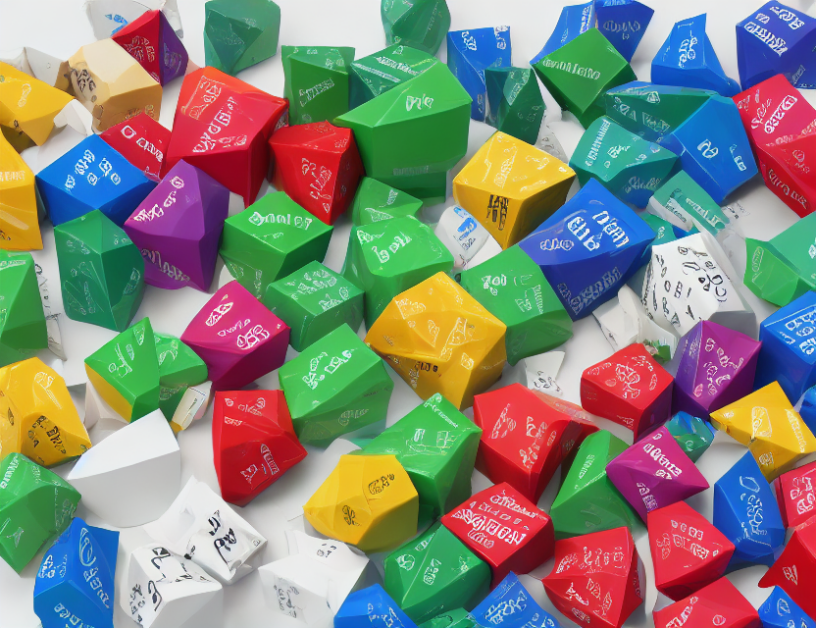