In this article, the authors propose a novel approach to extracting critical information from social media during disasters, using a technique called Few-Shot Learning. This method enables the system to learn and adapt quickly, even with limited training data, allowing it to accurately identify and categorize information related to casualties, damage, and other essential factors in a timely manner.
The authors explain that traditional methods of gathering disaster information rely on manual searches of global media outlets, which can be time-consuming and prone to errors. In contrast, Few-Shot Learning uses machine learning algorithms to quickly analyze social media posts and identify relevant information, such as the number of fatalities or injuries, and their locations.
The authors also highlight the advantages of using social media data during disasters. Unlike traditional media sources, which can be delayed or unreliable, social media provides real-time updates from people directly affected by the disaster. By leveraging this valuable information, emergency responders and aid organizations can make more informed decisions about how to allocate resources and prioritize their efforts.
To demonstrate the effectiveness of their approach, the authors present several case studies using data from recent disasters, such as the 2018 Papua New Guinea Earthquake and Hurricane Maria in 2017. In these cases, the Few-Shot Learning system was able to quickly identify and categorize critical information, providing valuable insights to emergency responders and aid organizations.
Overall, the authors’ proposed approach represents a significant advancement in the field of disaster response, enabling faster and more accurate gathering of essential information during crises. By leveraging social media data, emergency responders and aid organizations can make more informed decisions, ultimately leading to better outcomes for those affected by disasters.
Computation and Language, Computer Science
Fast and Accurate Estimation of Casualties in Disasters via Language Models
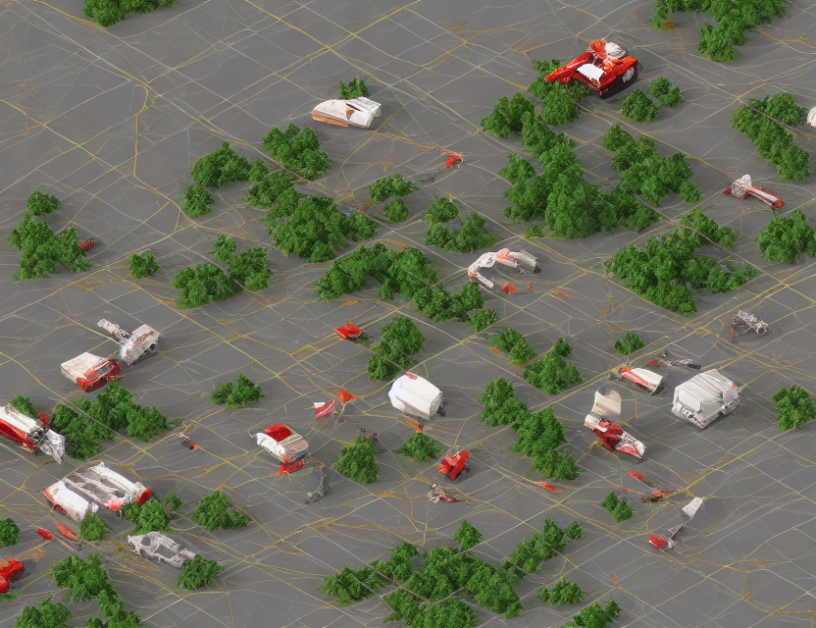