In this paper, researchers explored ways to enhance text-to-image synthesis by incorporating style transfer techniques. They proposed a novel approach that combines style transfer with textual inversion to create visually appealing and personalized images. The proposed method leverages the power of generative adversarial networks (GANs) to generate high-quality images based on given text prompts.
To achieve this, the authors first introduced a dataset of annotated images that represent various subjects, such as animals, vehicles, and objects. They then devised a novel training strategy that combines style transfer with textual inversion using GANs. The proposed method consists of two main components: (i) a style transfer module that transforms the input image based on the given style code, and (ii) an inversion module that modifies the output of the style transfer module to better match the input text.
The authors evaluated their approach using human evaluations, where raters assessed the quality and relevance of the generated images based on three factors: alignment with the given text, similarity to the object in the reference image, and naturalness of the image. The results showed that the proposed method outperformed existing approaches in terms of image quality and relevance to the input text.
To further demonstrate the effectiveness of their approach, the authors conducted a series of ablation studies to analyze the contribution of different components to the overall performance. They found that both style transfer and textual inversion play crucial roles in generating high-quality and personalized images.
In summary, this paper presents a novel approach for personalizing text-to-image synthesis with style transfer techniques. The proposed method leverages GANs to generate visually appealing and relevant images based on given text prompts, outperforming existing methods in terms of image quality and relevance. By combining style transfer with textual inversion, the authors were able to create a more effective and efficient way of generating personalized images that can be used in various applications, such as image generation, visual storytelling, and content creation.
Computer Science, Computer Vision and Pattern Recognition
Personalizing Text-to-Image Generation with Reinforcement Learning
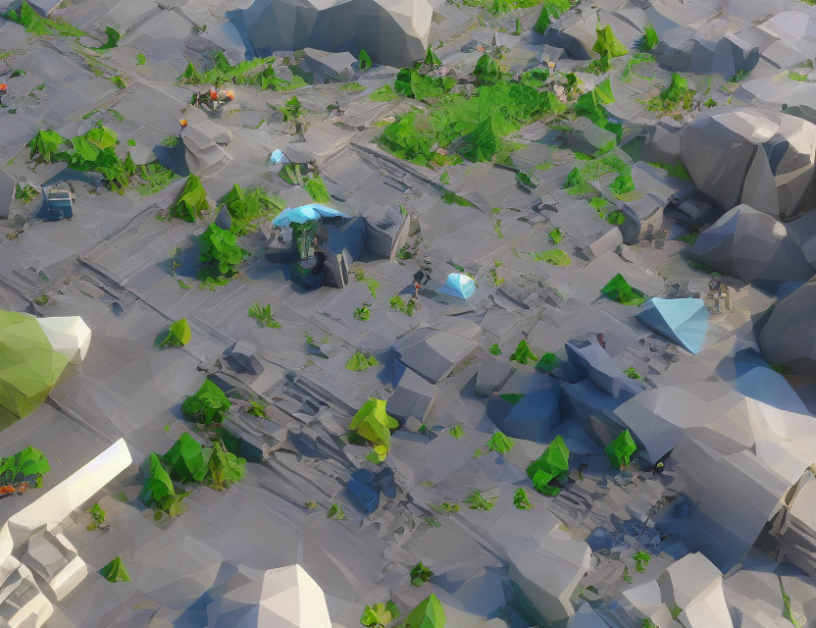