Linguistic typology and machine translation may seem like unrelated fields, but recent research has shown that combining them can significantly improve translation accuracy. In this article, we explore how bridging these two areas can lead to more accurate and efficient machine translation systems.
Context Matters
To start, it’s important to understand the context in which machine translation is used. Different languages have unique characteristics, and translating between them requires a deep understanding of each language’s grammatical structure, syntax, and idiomatic expressions. This is where linguistic typology comes in – by analyzing and categorizing languages based on their common features, researchers can develop more accurate machine translation systems.
Marrying Linguistic Pertinence with Data-Related Similarity
Our proposed method combines linguistic pertinence with data-related similarity to select the most appropriate auxiliary language for optimized translation outcomes. This approach ensures that the chosen language is not only grammatically similar to the source language but also has a high degree of similarity in terms of data distribution. By marrying these two aspects, we can create more effective and efficient machine translation systems.
Resilience Across Diverse Datasets
We assessed the resilience of our method across diverse datasets, demonstrating its ability to adapt to different language pairs and improving translation accuracy. This is crucial in real-world applications where machine translation systems are often tasked with translating between languages they have not seen before.
Fine-Tuning for Effective Translation
Finally, we discuss the importance of fine-tuning machine translation models to improve translation accuracy. Our proposed method leverages a large language model to raise a child in a way that enables effective and generalizable fine-tuning. By combining this approach with our linguistic typology-based method, we can achieve even better translation outcomes.
In conclusion, bridging linguistic typology and machine translation has the potential to significantly improve translation accuracy. By combining the strengths of both fields, researchers can create more effective and efficient machine translation systems that can adapt to diverse language pairs and data distributions. As the field continues to evolve, we can expect even more innovative approaches to emerge, leading to better translation outcomes for users around the world.
Computation and Language, Computer Science
Bridging Linguistic Typology and Multilingual Machine Translation with Multi-View Language Representations
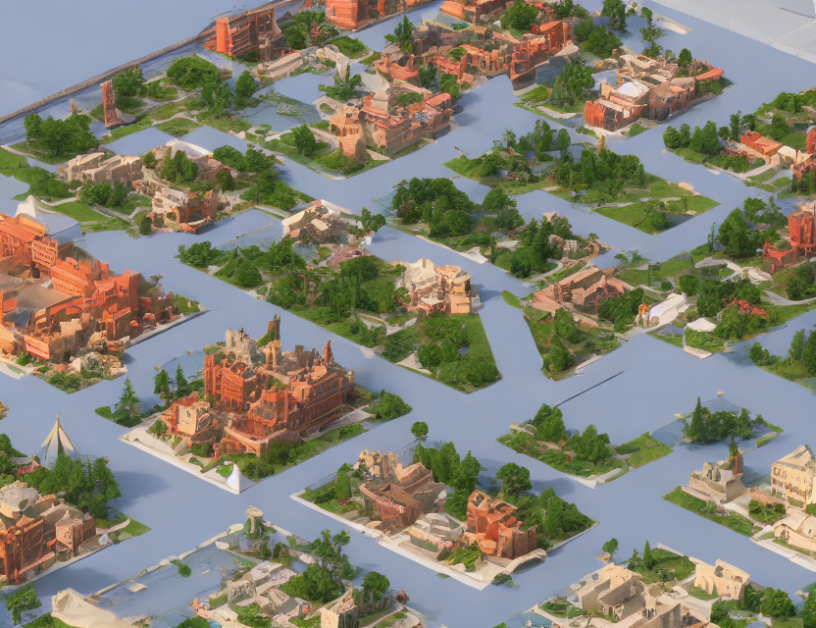