In this article, the authors propose a novel approach to generating fine-grained descriptions of human motions using a combination of diffusion models and ChatGPT-3.5. The proposed method, called Multi-Dimensional Diffusion Model for Motion Generation (MDM), is designed to overcome the limitations of traditional motion generation methods by providing more detailed and realistic descriptions of human movements.
The authors introduce a prompt strategy that guides the diffusion model to generate fine-grained descriptions based on different body parts, such as arms, legs, torso, neck, buttocks, and waist. They also propose an ablation study to evaluate the contribution of each module in the MDM framework.
The results show that the proposed method outperforms existing motion generation methods in terms of both objective evaluation metrics and human evaluations. The authors also conduct a generalization capability study to demonstrate the ability of MDM to generate descriptions for unseen motions, which shows promising results.
Overall, the article provides a significant contribution to the field of computer vision and machine learning by proposing a novel approach to generating fine-grained descriptions of human motions. The proposed method has important implications for applications such as virtual reality, robotics, and motion capture.
Computer Science, Computer Vision and Pattern Recognition
Fine-Grained Motion Generation via Textual Descriptions: A Survey
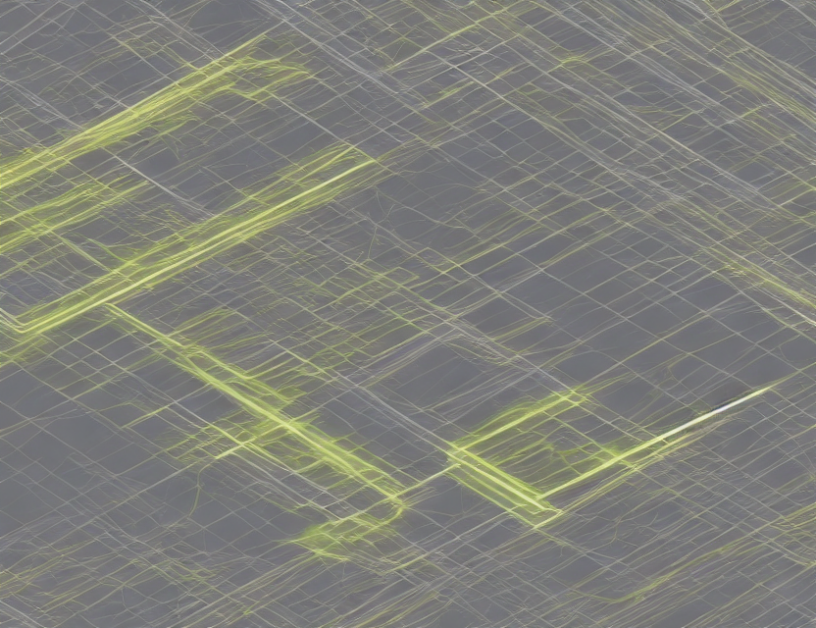