- Reranking can significantly improve the quality of search results for biomedical texts.
- Incorporating hard negatives into the prompt decoding process can enhance the effectiveness of the reranker, especially when using a smaller model size.
- The choice of first-stage retrieval model and the number of candidates considered for reranking are crucial factors that impact overall effectiveness.
- Evaluating rerankers over different first-stage retrievers is essential to capture nuances in a manner free of confounders.
- Data augmentation can potentially improve the performance of rerankers.
Computer Science, Information Retrieval
Improving Cross-Encoder Effectiveness for Reranking in Information Retrieval
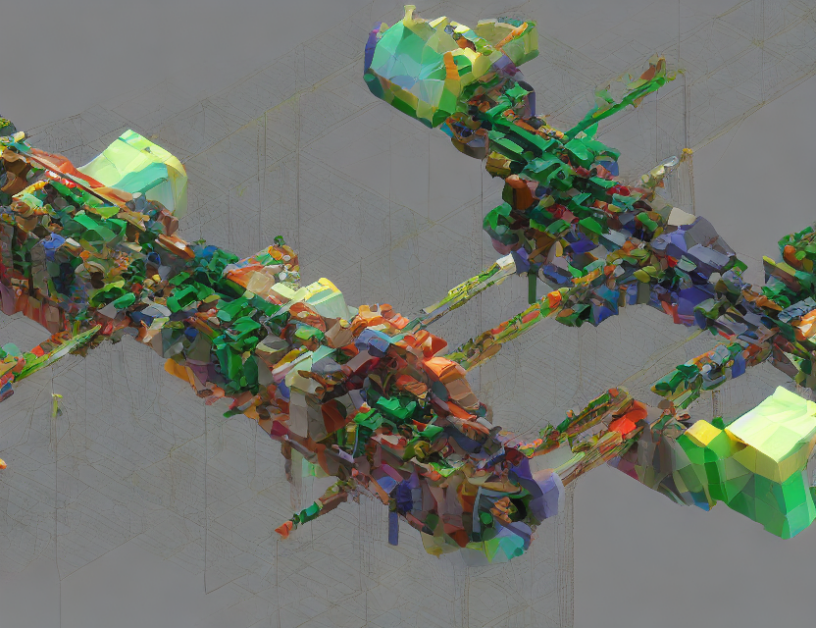