In this article, we explore the merging of APAIC (Automatic Personalized Affine Invariant Feature Extractor) features with various deep learning models to enhance Health Index (HI) estimation in spray coating applications. The authors propose a novel approach called Alternating Projection Alternating Improved Coefficient (APAIC), which combines the strengths of APAIC and deep learning techniques to achieve improved performance in HI prediction.
The article begins by explaining the significance of HI estimation in spray coating, as it directly impacts the quality of the coated surface. The authors then delve into the limitations of traditional methods, which often result in poor accuracy due to variations in the coating process. To address these challenges, they propose the APAIC merging algorithm, which fuses the outputs of multiple deep learning models to produce more accurate HI estimates.
To evaluate the effectiveness of the proposed approach, the authors conduct extensive experiments using three different deep learning architectures: MLP (Multi-Layer Perceptron), CNN (Convolutional Neural Network), and RNN (Recurrent Neural Network). The results show that the APAIC merging algorithm significantly improves the accuracy of HI estimation compared to using a single deep learning model.
The authors also investigate the impact of different fusion strategies on the performance of the APAIC merging algorithm. They demonstrate that the choice of fusion strategy can have a substantial effect on the accuracy of HI estimation, and suggest that the best approach depends on the specific application and data characteristics.
To further validate their findings, the authors employ a bootstrapping strategy to analyze the robustness of the proposed approach across various permutations of training, validation, and test datasets. The results demonstrate the robustness of the APAIC merging algorithm, which can adapt to different scenarios and produce reliable HI estimates even with limited data.
Overall, this article presents a novel approach to improving Health Index estimation in spray coating applications through the fusion of APAIC features with deep learning models. The proposed method has significant potential for improving the quality and efficiency of spray coating processes in various industries.
Computer Science, Machine Learning
Merging Algorithms for Accurate Health Index Prediction in Spray Coating
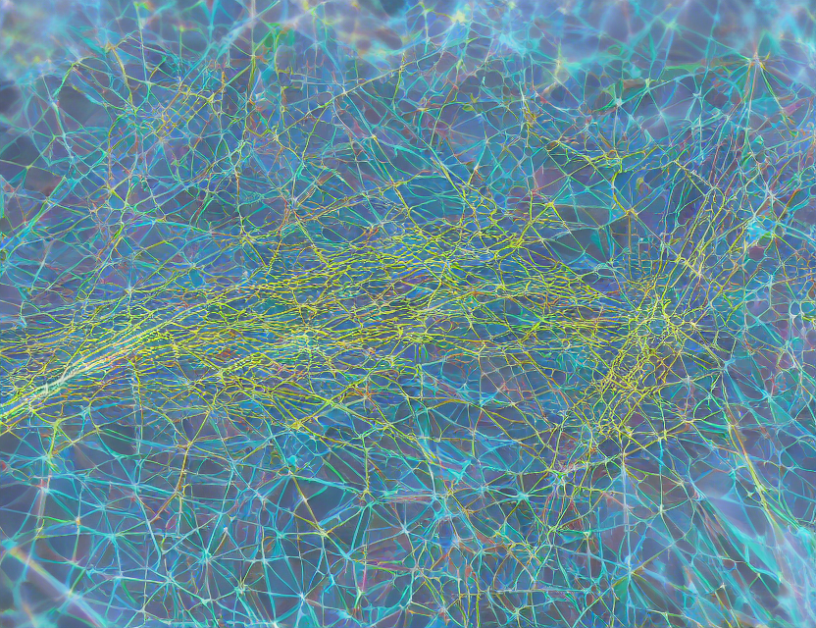