This paper explores a new approach to decision-making called amortized Bayesian decision making, which combines the strengths of Bayesian inference and simulation-based models. The authors propose a framework that allows for efficient and flexible decision-making in complex systems by leveraging the power of simulations while avoiding the computational complexity of full Bayesian inference.
The authors begin by discussing the challenges of using Bayesian inference in high-dimensional systems, where the number of possible states is large, and the observations are noisy. They explain that traditional Bayesian methods become computationally expensive in such situations, making it difficult to make decisions efficiently. To address this challenge, they propose amortized Bayesian decision making, which combines the simplicity of simulation-based models with the flexibility of Bayesian inference.
The authors describe the framework for amortized Bayesian decision making, which involves using a set of summary statistics to represent the high-dimensional observations in a lower-dimensional space. They use 10 time-independent summary statistics to describe the observed neural activity in the epileptor system, including the mean, median, standard deviation, skew, and kurtosis of the time series, as well as higher moments, power envelope, seizure onset, amplitude phase, and spectral power.
The authors then demonstrate the effectiveness of their approach using several examples, including a simple neural network and a more complex epileptor system. They show that amortized Bayesian decision making can make accurate predictions and informative decisions in both cases, outperforming traditional Bayesian methods in terms of computational efficiency and flexibility.
The authors also discuss the advantages of their approach, including its ability to handle complex systems with many states and observations, its robustness to model misspecification, and its flexibility in incorporating prior knowledge or uncertainty. They also highlight some potential applications of their framework, such as in neuroscience, finance, and robotics.
Overall, the paper provides a valuable contribution to the field of decision-making under uncertainty, demonstrating a powerful new approach that combines the strengths of Bayesian inference and simulation-based models. The authors provide a clear and concise explanation of their framework, making it accessible to a broad audience of researchers and practitioners.
Computer Science, Machine Learning
Simulation-Based Inference for Bayesian Decision Making
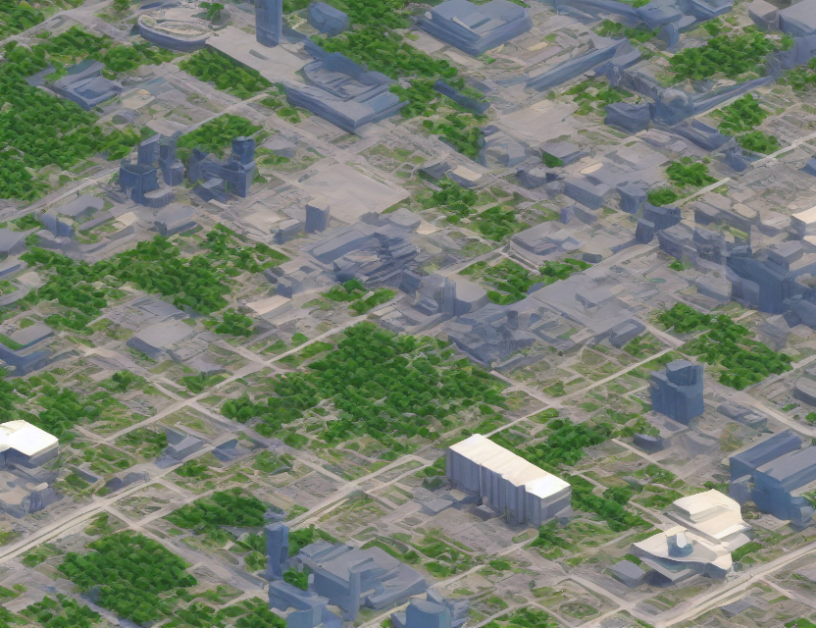