In simple terms, LLMs are like fast food restaurants that can churn out text quickly but may not provide nutritious or accurate information. They can be useful for generating initial ideas or summarizing large amounts of data, but they should not replace human judgment and critical thinking in scientific communication. Just as we would not rely solely on fast food for sustenance, we should not rely solely on LLMs for our scientific understanding.
The authors suggest that researchers must develop strategies to assess the quality and accuracy of LLM-generated text before using it in their work. This may involve defining clear criteria for evaluating AI-related content and implementing verification methods to ensure its accuracy. While this may take additional time and effort, it is essential to maintain the integrity and reliability of scientific information in today’s fast-paced research environment.
Ultimately, the article cautions against relying solely on LLMs for scientific communication, emphasizing the importance of balancing efficiency with accuracy and critical thinking. By using LLMs as a starting point rather than a substitute for human judgment, scientists can harness their potential while avoiding common pitfalls in AI-mediated communication.
Computation and Language, Computer Science
The Hidden Truth Behind Large Language Models in Scientific Publishing
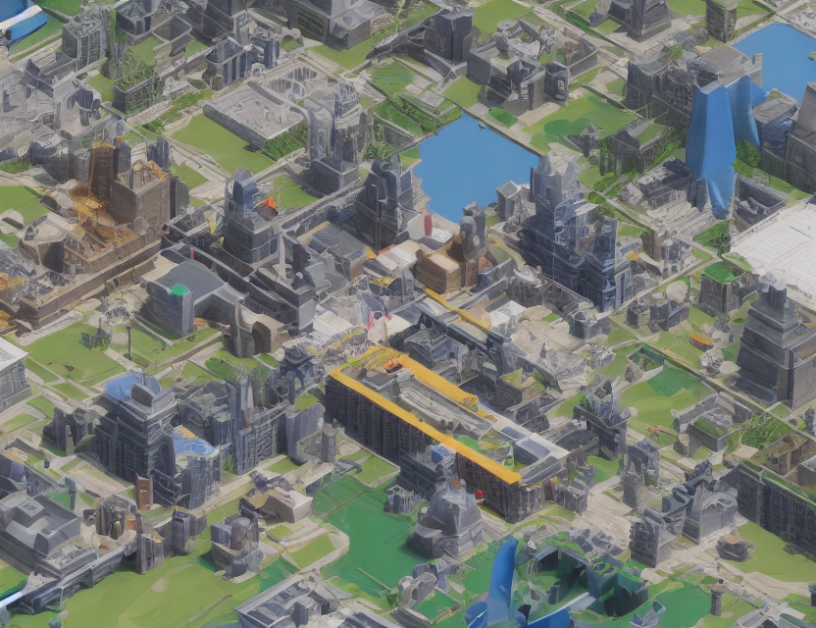