In recent years, artificial intelligence has made significant progress in generating images, leading to transformative changes across various applications. One area of interest is the generation of human images, which has gained significant attention due to its wide applicability and popularity. To address this challenge, researchers have proposed a novel approach called "Multi-Identity Synthesis," which enables the generation of images that preserve the identity of the subject.
The authors’ primary focus is on preserving human identity during the image generation process. They achieve this by introducing a new cross-attention mechanism that allows the model to differentiate between multiple identities in an image. This enhancement enables the generation of images with diverse styles and poses while maintaining the subject’s identity.
The proposed approach is based on a modified StableDiffusion model, which is a type of text-to-image diffusion model. The model includes both trainable and frozen modules to improve the image quality and preserve the subject’s identity. The authors also introduce a novel training strategy that enables the model to learn from a small number of images, making it easier to train.
To demonstrate the effectiveness of their approach, the authors conduct experiments using several datasets. The results show that their method outperforms existing methods in terms of image quality and ability to preserve the subject’s identity.
The key insight behind this work is the recognition that human identity is a critical aspect of image generation. By developing a model that can differentiate between multiple identities, the authors have opened up new possibilities for image generation with multi-identity synthesis. Their approach has far-reaching implications, including applications in advertising, entertainment, and virtual reality.
In summary, this article presents a novel approach to image generation that preserves the identity of the subject. By introducing a cross-attention mechanism that can differentiate between multiple identities, the authors have enabled the generation of images with diverse styles and poses while maintaining the subject’s identity. Their work has significant implications for various applications, including advertising, entertainment, and virtual reality.
Computer Science, Computer Vision and Pattern Recognition
Tuning-Free Image Synthesis and Editing Techniques: A Survey
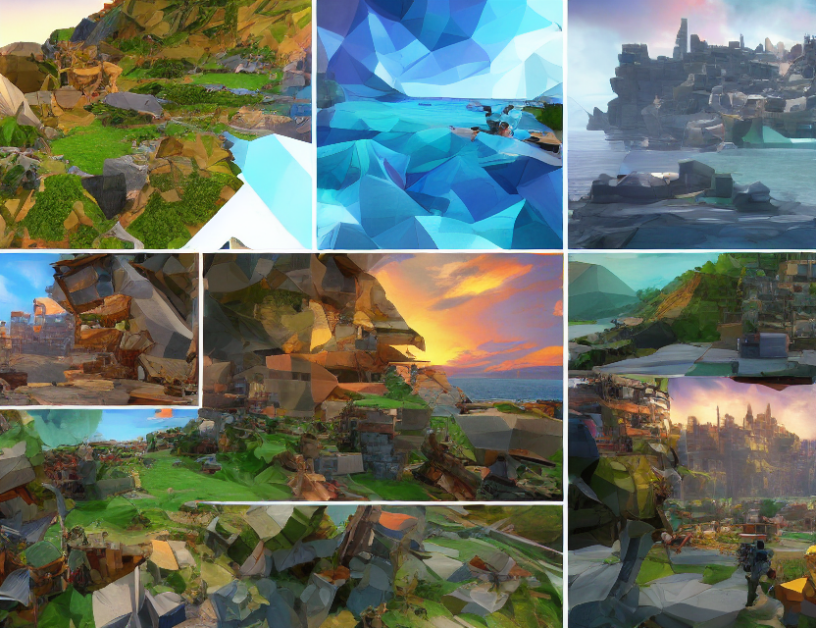