In this study, researchers aimed to investigate the impact of internet data on language models’ performance and bias. They analyzed a dataset provided by HuggingFace, which contained 99442 samples, and split it into three non-overlapping batches – reference (2000 sentences), clean (2000 sentences), and anomalous (3642 sentences). The researchers then used the Open Pre-trained Transformer (OPT) language model to analyze these datasets and evaluate the models’ performance.
The researchers found that the language model’s performance improved with more data, but it also became more biased towards certain groups of people. They observed that the model was more accurate when it came to sentences containing words related to Western cultures, such as "Europe" or "China," compared to those related to non-Western cultures like "India" or "Africa." This bias was particularly noticeable in the anomalous batch of data.
The researchers also found that the model’s performance varied depending on the size of the training data. They discovered that smaller datasets resulted in less accurate predictions, but larger datasets improved the model’s accuracy significantly. However, they noted that larger datasets can also lead to a higher risk of bias and safety concerns due to the diversity and unfiltered nature of the internet data used to train the model.
To address these issues, the researchers suggest using additional techniques to fine-tune the pre-trained language models, such as adversarial training or reinforcement learning. They emphasize the importance of balancing accuracy with bias and safety concerns in AI language models, particularly when dealing with sensitive topics like race, ethnicity, or nationality.
In summary, this study highlights the potential risks associated with using large datasets to train language models, particularly those that are unfiltered and diverse. The researchers emphasize the need for caution when evaluating these models’ performance and bias, as larger datasets can lead to a higher risk of bias and safety concerns. They suggest using additional techniques to fine-tune the pre-trained language models to improve their accuracy while minimizing bias and safety risks.
Computer Science, Machine Learning
Unmasking Bias in AI-Generated Text: A Study on Human Perception of Nationalities
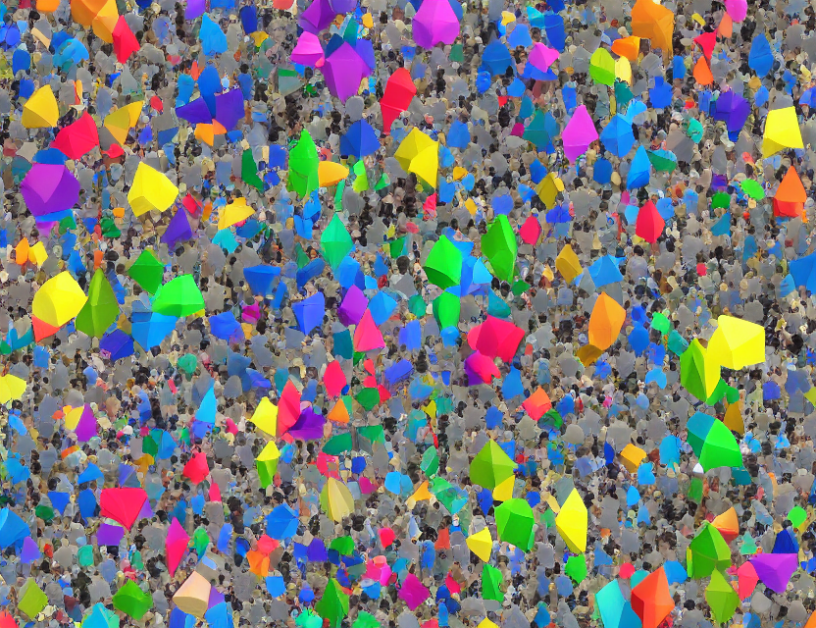