In the quest for efficient research, automating peer review has emerged as a pivotal solution. This process involves evaluating and providing feedback on research papers by experienced scientists, typically through a manual effort-intensive process. However, with advancements in language models (LLMs), we can now leverage these models to automate this crucial aspect of the research cycle. By doing so, we can significantly accelerate the research process while maintaining its quality and rigor.
Firstly, it is essential to appreciate that peer review is not just a mechanical evaluation of research papers but rather an opportunity to gain valuable insights into the research process itself. As Krenn et al. (2015) highlight, even without understanding the underlying theories or hypotheses, automated machines can still make discoveries. However, facilitating scientific understanding in humans through these machines demands more than just technical prowess; it requires a deeper appreciation of the research process itself. Therefore, when automating peer review, we must consider not only the technical aspects but also the broader context of how research works.
To achieve this goal, we need to identify what is needed to foster scientific understanding in humans through automated peer review. According to Xu et al. (2023), exploring and verbalizing academic ideas by concept co-occurrence can help facilitate this process. By using language models that are capable of capturing the nuances of complex research concepts, we can create a more comprehensive understanding of the research process. Additionally, Yi Xu et al. (2023) propose the use of large language models to automate open-domain scientific hypotheses discovery, which can help identify potential areas of research that may benefit from human evaluation.
However, peer review is not just about evaluating research papers; it is also a critical component of the broader research ecosystem. As Maynez et al. (2020) highlight, peer review is a universal practice across various research fields, and automating this process can significantly contribute to the development of a general research agent applicable in multiple disciplines. Moreover, peer review predominantly involves textual analysis, which does not require extensive digital interactions or physical experiments, making it an ideal candidate for early-stage automation.
Finally, peer review provides valuable insights into how humans assess subjective aspects like the significance of a research question. By analyzing these value judgments explicitly through automated peer reviews, we can begin to demystify the evaluative process in research and identify areas where human values align with machine learning models. Moreover, understanding how humans evaluate research questions is crucial, especially considering that alignment with human values is a significant challenge.
In conclusion, automating peer review has the potential to revolutionize the research landscape by accelerating the evaluation process while maintaining its quality and rigor. By leveraging LLMs to automate this critical aspect of the research cycle, we can gain valuable insights into the research process itself, identify areas where machine learning models can enhance human evaluations, and align machine learning models with human values more effectively. As we embark on this exciting journey towards automating peer review, let us remember that it is not just about technological advancements but also about understanding how humans evaluate and assess research. By taking a holistic approach to automating peer review, we can unlock the full potential of AI in research and accelerate scientific progress towards a brighter future.
Artificial Intelligence, Computer Science
Advancing Research Autonomy: Navigating the Challenges of Machine-Generated Research Questions
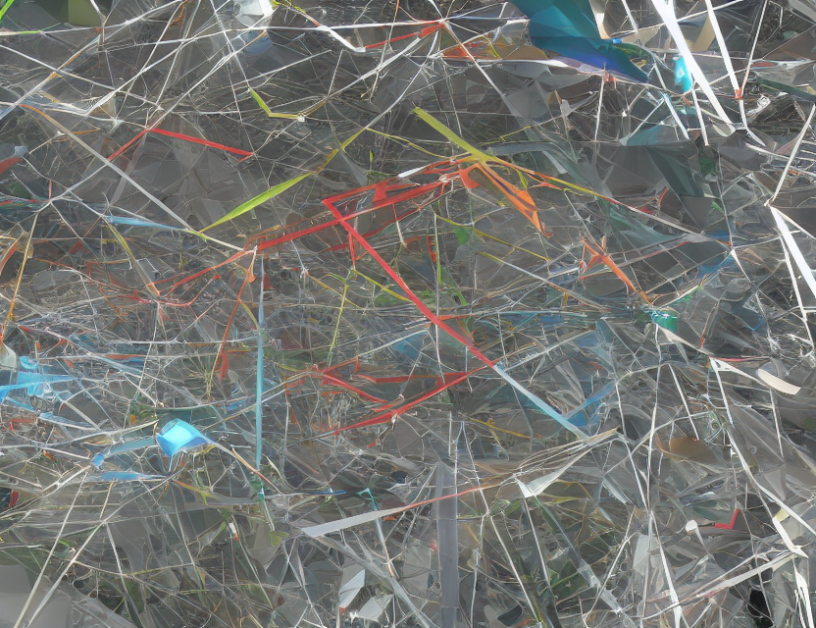