In this research paper, the authors aim to address the issue of class imbalance in few-shot learning (FSL) tasks, where the model is trained on a small number of labeled examples from the minority class. To overcome this challenge, they propose a novel approach called SYNC-CLIP, which leverages both real and synthetic data to improve the model’s generalization capability.
The authors begin by highlighting the limitations of existing FSL methods that rely solely on real data, as these approaches often fail to capture the domain-specific information within the minority class. To address this issue, SYNC-CLIP incorporates domain-specific prompts and shared visual prompts to align the cross-domain features and enable the model to learn from both real and synthetic data.
The authors demonstrate the effectiveness of SYNC-CLIP through extensive experiments on various open-vocabulary and cross-domain datasets. The results show that SYNC-CLIP outperforms existing FSL methods and achieves a more balanced performance between base and novel classes. Additionally, the authors show that SYNC-CLIP can handle complex tasks such as zero-shot learning (ZSL) and generic zero-shot learning (GZSL), where the model is required to classify unseen classes without any labeled data from those classes.
To further illustrate the effectiveness of SYNC-CLIP, the authors provide a detailed analysis of the impact of synthetic data on the model’s performance. They show that incorporating domain-specific prompts and shared visual prompts can significantly improve the model’s ability to generalize to unseen classes, especially in the ZSL setting.
In conclusion, SYNC-CLIP represents a significant advancement in the field of FSL, as it demonstrates the potential to overcome class imbalance issues through the judicious use of both real and synthetic data. By leveraging domain-specific prompts and shared visual prompts, SYNC-CLIP can learn from both domains and classes, leading to improved generalization performance and a more equitable balance between base and novel classes.
Computer Science, Computer Vision and Pattern Recognition
Alleviating Imbalance in Visual Classification via Synthetic Data Augmentation
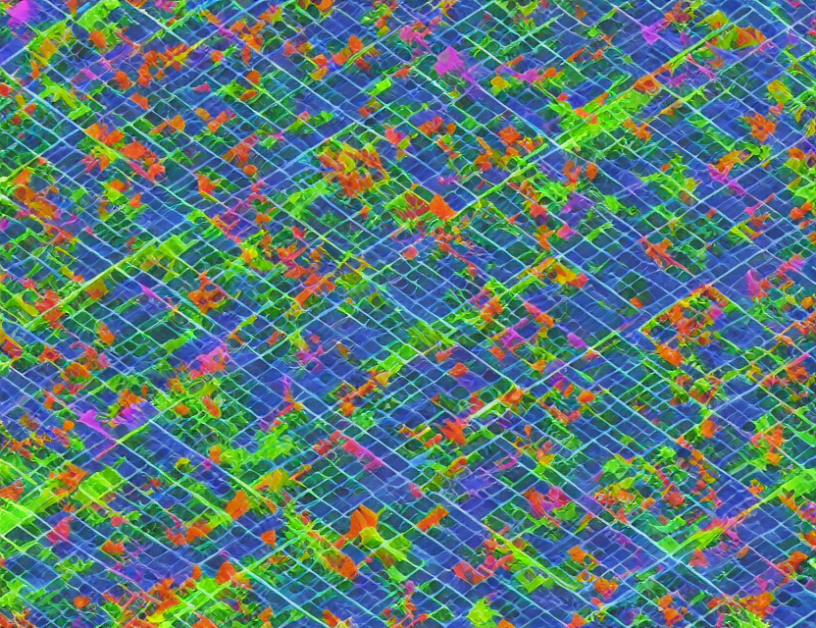