In this article, researchers explore a new method for completing 3D scenes from semantic occupancy grids. The proposed approach leverages a combination of depth estimation and graph-based techniques to efficiently model large-scale contexts. By identifying non-empty regions through depth estimation and focusing on specific areas, the method reduces computational complexity without sacrificing accuracy.
The researchers evaluate their approach using several datasets and compare it to existing methods. The results show that their method outperforms others in terms of both accuracy and efficiency. They also demonstrate the effectiveness of their approach through visualization experiments.
The authors highlight the importance of determining the optimal number of queries with different granularities, which should align with the statistical distribution of object granularity within the scene. This ensures that queries can effectively handle semantic regions corresponding to their specific granularity levels.
In summary, this article presents a novel method for completing 3D scenes from semantic occupancy grids, which leverages depth estimation and graph-based techniques to efficiently model large-scale contexts. The proposed approach demonstrates improved accuracy and efficiency compared to existing methods, making it a promising solution for applications in computer vision and robotics.
Computer Science, Computer Vision and Pattern Recognition
Deep Learning for 3D Scene Completion: A Comprehensive Review
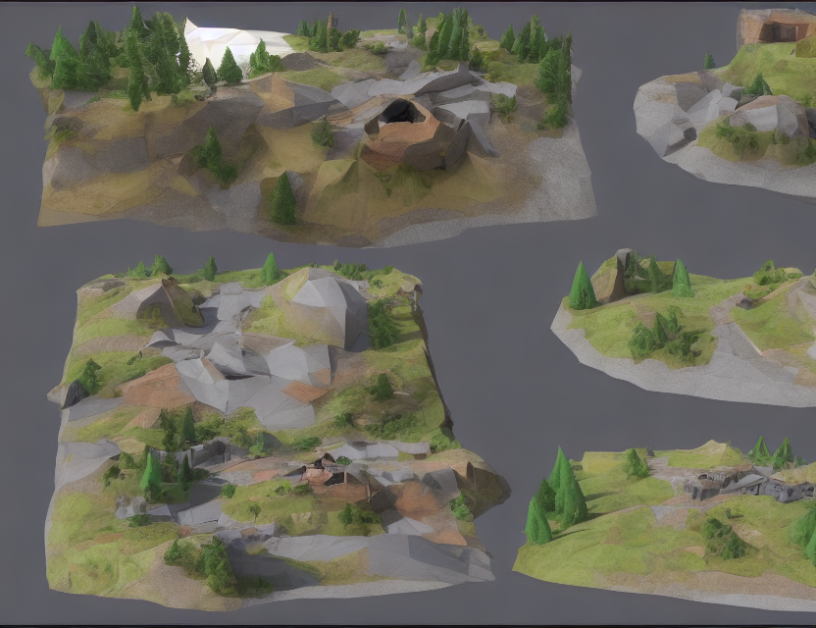