In this article, we delve into the realm of deep learning (DL) and its potential to revolutionize wireless communications. DL is a subset of machine learning that involves neural networks with multiple layers, allowing them to learn complex patterns in data. By leveraging these techniques, researchers aim to improve the performance of wireless communication systems in various ways.
Firstly, we explore unsupervised learning algorithms like k-means clustering, which can help discover hidden relationships in data without any prior knowledge of labels. These techniques are particularly useful when dealing with large datasets, such as those encountered in IoT applications.
Next, we turn our attention to deep learning (DL) and its potential to enhance wireless communication systems. DL algorithms can be trained in both supervised and unsupervised manners, depending on the availability of labeled data. By leveraging these techniques, researchers have demonstrated improved system performance, reduced error floors, and enhanced detection capabilities.
To further illustrate the potential of DL, we examine the impact of distance between tags and readers on detection performance. We also discuss the benefits of using antenna selection schemes to optimize reader BER (Bit Error Rate).
Finally, we provide a concise overview of the article’s key takeaways, highlighting the potential of DL to enhance wireless communication systems. By leveraging these techniques, researchers can improve system performance, reduce errors, and enhance detection capabilities. As the field continues to evolve, we can expect even more exciting developments in the realm of deep learning for wireless communications.
Computer Science, Information Theory
Directions for Future Research in Machine Learning
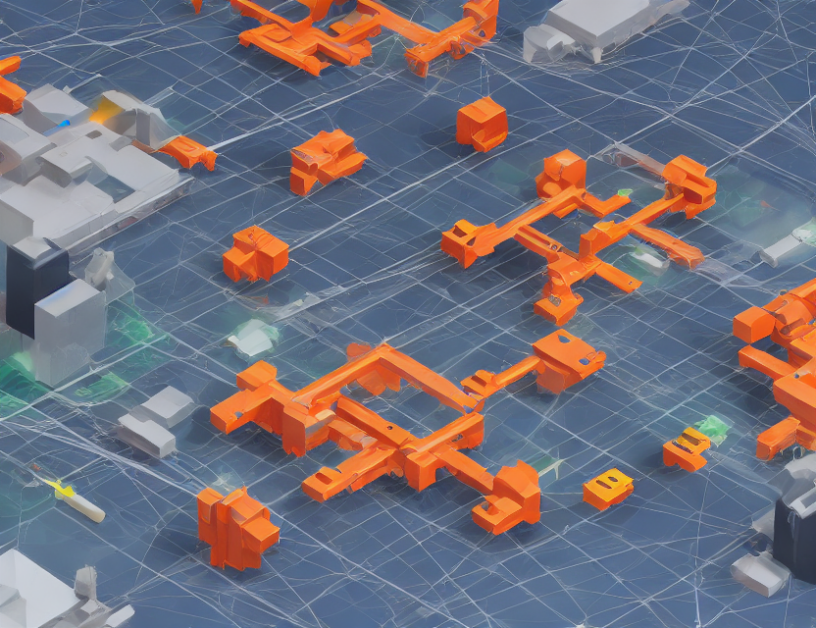