In this article, we dive into the exciting field of zero-shot novel view synthesis, a technique that enables generating 3D objects from single in-the-wild images. We explore how recent advances in diffusion models and pre-training have enabled the creation of high-quality 3D objects without requiring additional training data or annotations.
Imagine having a magic wand that could transform any random image into a 3D object! Zero-shot novel view synthesis makes this possible by leveraging pre-trained diffusion models, fine-tuned on large datasets like Objaverse, to generate images of an object from a single input image.
The challenge lies in the ambiguity of single in-the-wild images, which can lead to uncontrollable results for particular object categories. To overcome this, researchers have proposed various methods that distill 2D image priors into 3D representations, achieving remarkable text-to-3D object generation. However, these methods face the Janus problem when encountering real 3D datasets, making it challenging to generate high-quality 3D object shapes.
To tackle this issue, researchers have proposed DreamFusion-like methods that utilize similar distillation approaches to execute image-to-3D tasks. These methods demonstrate remarkable capabilities in generating high-quality 3D objects from single in-the-wild images without requiring additional training data or annotations.
In summary, zero-shot novel view synthesis has revolutionized the field of 3D object generation by enabling the creation of high-quality 3D objects from single in-the-wild images. By leveraging pre-trained diffusion models and fine-tuning them on large datasets, researchers have been able to generate images of an object from a single input image with remarkable accuracy. However, challenges remain in generating high-quality 3D objects without encountering real 3D datasets, and researchers continue to develop innovative methods to overcome these challenges.
Computer Science, Computer Vision and Pattern Recognition
DreamComposer: Elevating Diffusion Models for Novel View Synthesis
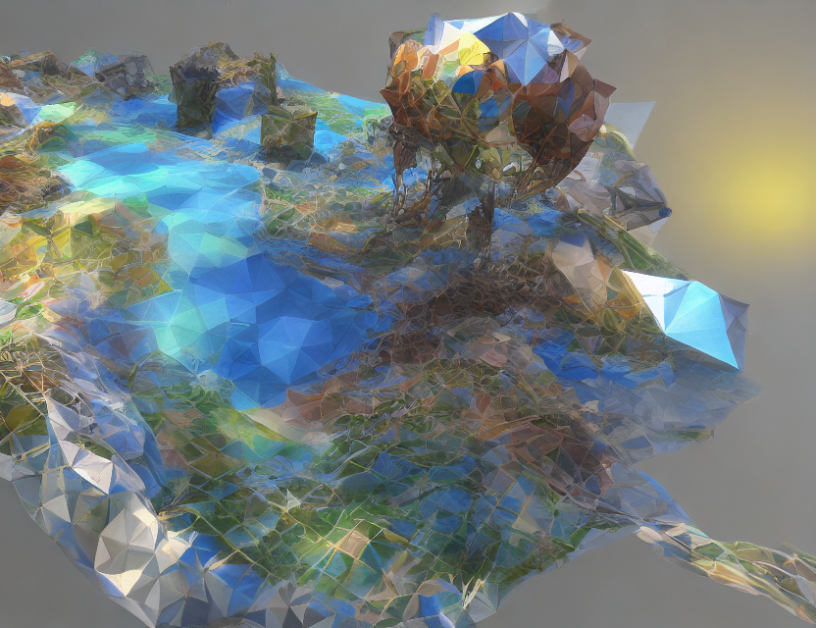