In recent years, there has been growing interest in addressing fairness and discrimination in machine learning applications, particularly in healthcare. The article "Translating Intersectionality to Fair Machine Learning in Health Sciences" by E. Lett and W. G. La Cava (2023) provides a valuable contribution to this field by exploring the concept of intersectionality and its application to fair machine learning in health sciences.
Intersectionality is a social justice framework developed by Kimberlé Crenshaw that emphasizes the importance of considering multiple forms of discrimination simultaneously, such as racism, sexism, homophobia, and classism. In the context of machine learning, intersectionality can help identify and address biases in algorithms that may disproportionately affect marginalized groups.
The authors propose a framework for translating intersectionality to fair machine learning in health sciences, which involves three main steps: (1) conceptualizing intersectionality, (2) estimating fairness metrics, and (3) optimizing fairness-aware models. They also discuss several challenges and limitations of this approach, including the need for high-quality data and the potential for unintended consequences.
To illustrate their framework, the authors use a case study on predicting cardiovascular disease risk in African American and white populations. They show how intersectionality can help identify biases in the model and improve its fairness by considering multiple sensitive attributes simultaneously.
Throughout the article, the authors use clear and concise language to explain complex concepts, making it accessible to a wide range of readers. They also provide several analogies and metaphors to help demystify the technical terms, such as comparing the estimation of fairness metrics to "playing a game of poker" or "tuning a piano."
In summary, "Translating Intersectionality to Fair Machine Learning in Health Sciences" is an important article that contributes to the growing body of research on fair machine learning. By providing a framework for applying intersectionality to healthcare applications, the authors demonstrate how this approach can help identify and address biases in algorithms that may disproportionately affect marginalized groups.
Computer Science, Machine Learning
Fairness in Machine Learning: A Survey of Approaches and Challenges
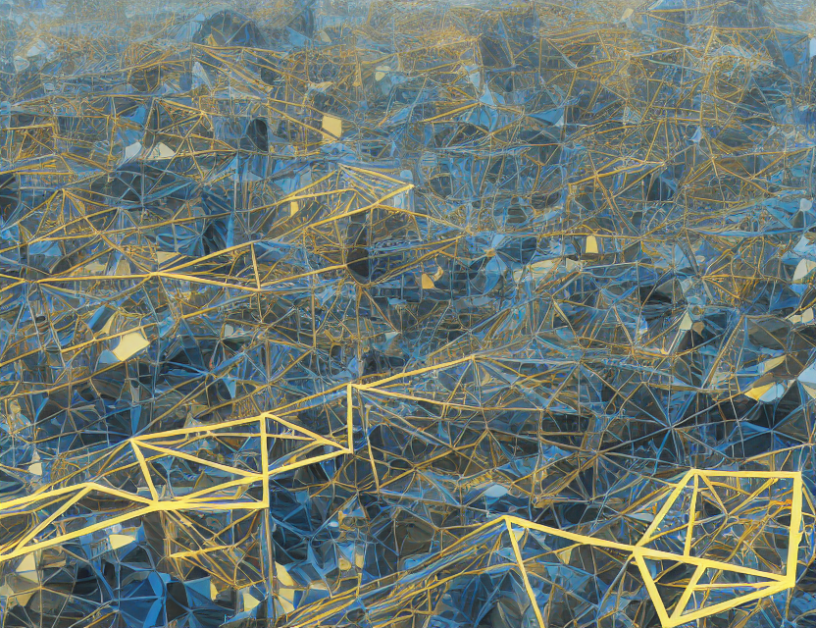