In this paper, the authors aim to improve the performance of CLIP (Content-Aware Image Captioning) by introducing a new approach called Alpha-CLIP. The main idea is to focus on the regions of interest in an image instead of processing the entire image, which can help the model understand and reason about the content more accurately.
To achieve this, Alpha-CLIP uses a novel method called "masking" that allows the model to specify the region of interest by applying a mask or a box around the desired area. This approach is different from traditional CLIP, which processes the entire image without any spatial attention.
The authors tested their approach on several benchmark datasets and achieved competitive results compared to other state-of-the-art models. They also demonstrated that Alpha-CLIP can generate more accurate captions by focusing on the relevant regions of the image.
In simple terms, Alpha-CLIP is like a magnifying glass for images. It helps the model focus on specific parts of the image instead of looking at the whole thing, which can lead to more accurate and detailed captions. This approach has the potential to improve the performance of image captioning models in various applications such as image retrieval, object detection, and accessibility for visually impaired individuals.
The authors also introduced a new method called "SAM" (Scene Adaptation Model) that can adapt to different scenes and objects in an image by learning from a large dataset of images with diverse content. This approach can help improve the generalization ability of CLIP and other image captioning models, leading to better performance in various applications.
Overall, the authors’ approach improves upon traditional CLIP by introducing spatial attention mechanisms that allow the model to focus on specific regions of interest, leading to more accurate and detailed captions. This can have significant implications for applications such as image retrieval, object detection, and accessibility for visually impaired individuals.
Computer Science, Computer Vision and Pattern Recognition
Improved Understanding through Focused Attention: Enhancing CLIP with Alpha-CLIP and Masking.
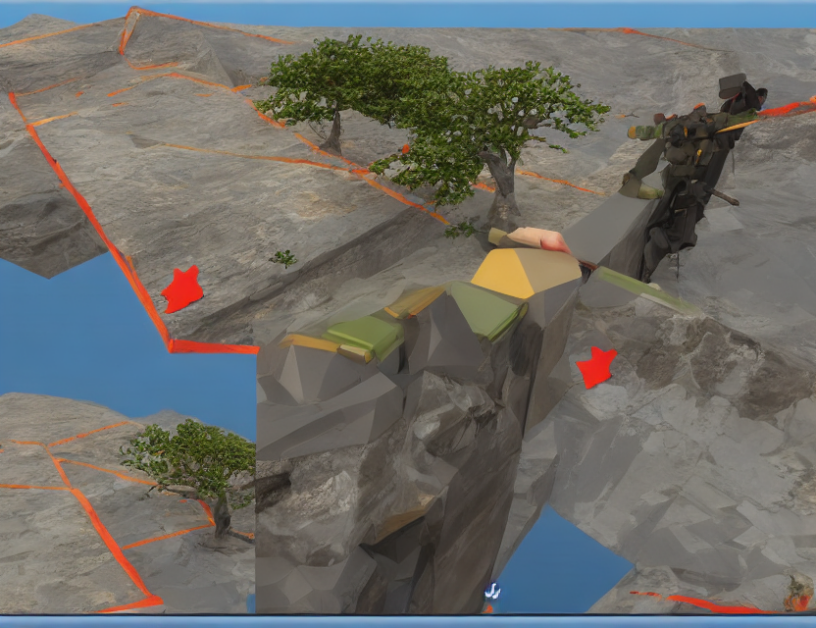