Surgical feedback is crucial for improving surgical skills, but manual transcription of feedback from audio is time-consuming and expensive. To address this challenge, researchers proposed a deep multimodal fusion model that combines manual and automated transcriptions to improve the accuracy of surgical feedback classification.
The proposed model uses a joint ensemble of manual and automated transcriptions to create a more comprehensive understanding of the surgical feedback. The authors experimented with different fusion strategies, including joint feature and staged feature fusion, and found that joint ensemble fusion outperformed other strategies. They also compared the performance of manual and automated transcription and found that manual transcription offered better results, but was more time-consuming and expensive.
The authors evaluated their model on a dataset collected from surgical feedback videos and achieved promising results. They demonstrated that their model could accurately classify surgical feedback into different categories, such as praise or criticism, with an F1 score of 84.68%. They also showed that their model outperformed other state-of-the-art models in the literature.
The researchers acknowledge some limitations of their study, including the small sample size and the lack of diversity in the datasets used for training and testing. However, they argue that their approach has the potential to improve the efficiency and accuracy of surgical feedback classification, which could have significant implications for surgical education and training.
In summary, this article proposes a deep multimodal fusion model that combines manual and automated transcriptions to improve the accuracy of surgical feedback classification. The proposed model outperforms other state-of-the-art models and has the potential to revolutionize surgical education and training.
Computer Science, Machine Learning
Improving Surgical Feedback Classification with Deep Multimodal Fusion
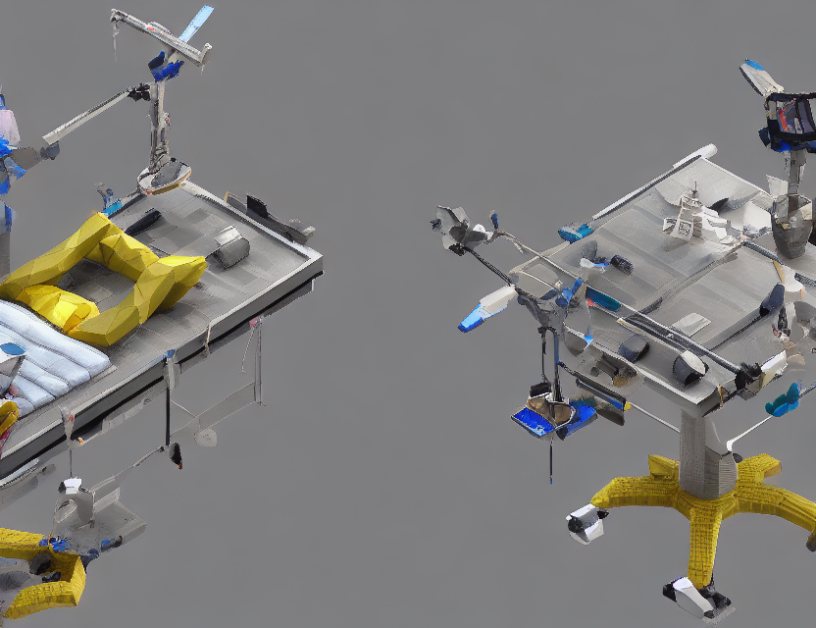