In this article, we compare various image-to-image translation methods for video editing. These methods aim to convert a source image into a target image with desired changes, such as changing the background or adding objects. We evaluate these methods using 20 prompts and corresponding generated images in different styles and contents.
To achieve comprehensive evaluation, we use various metrics that measure different aspects of image quality, including depth of field, masterpiece, best quality, and more. Our results show that some methods perform better than others depending on the specific task and input image. For instance, Civitai’s method outperforms others in generating images with detailed and realistic content, while Gen-2’s method is excellent at creating images with diverse styles.
One of the challenges in image-to-image translation is dealing with the complexity of video editing tasks, which often require manipulating multiple frames or objects in a sequence. To address this challenge, we propose a new approach called "null-text inversion," which uses guided diffusion models to invert the text-to-image process. This approach allows for more accurate and efficient image editing, especially when dealing with complex tasks such as changing multiple objects in a scene.
Another important consideration in video editing is the use of attentions, which help the model focus on specific parts of the input image when generating the output. We explore the effectiveness of using attentions in our evaluated methods and find that they significantly improve the quality of generated images.
In summary, this article provides a comprehensive comparison of various image-to-image translation methods for video editing, highlighting their strengths and limitations. By proposing new approaches such as null-text inversion and exploring the use of attentions, we demonstrate the potential of these methods for improving the quality and efficiency of video editing tasks.
Computer Science, Computer Vision and Pattern Recognition
Mastering Video Editing with Latent Diffusion Models
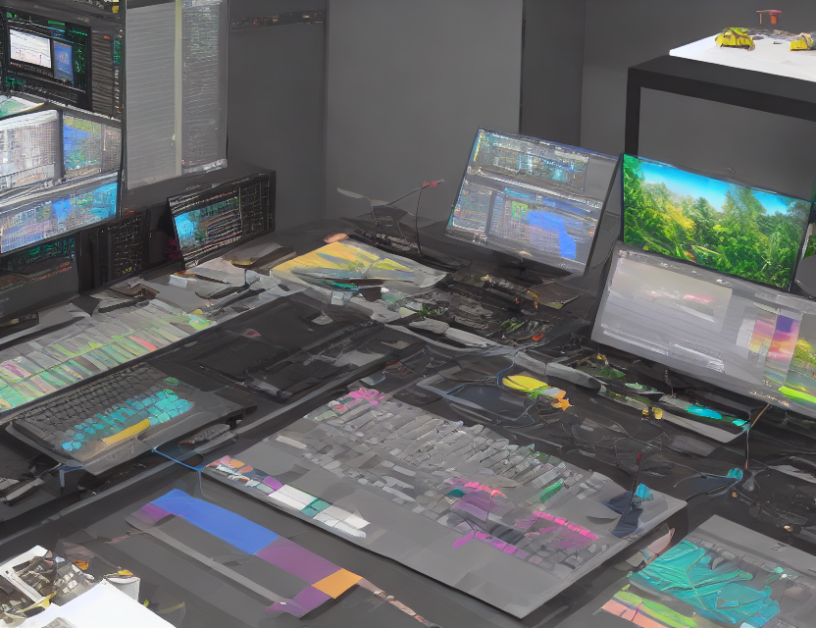