In this article, Don R Swanson, Neil R Smalheiser, and Vetle I Torvik explore the importance of medical subject headings (MeSH) in literature-based discovery. They discuss how ranking indirect connections between MeSH terms can help identify relevant biomedical concepts. The authors explain that traditional methods of linking concept associations rely on manual annotation or automated techniques that only consider direct connections between terms. However, this approach overlooks the potential connections between related but not directly linked terms.
To address this limitation, the authors propose a methodology based on the lowest common denominator approach, which discards concepts without UMLS representations and associations not appearing in PubMed abstracts. Despite this constraint, their method still extracts a significant number of concept associations for quantitative analysis. The experiment reveals interesting results, highlighting differences in performance between models when comparing different data sources.
The authors also compare their approach to other link prediction systems, including AGATHA and Node2Vec. They find that incorporating MeSH representations improves the accuracy of link predictions, demonstrating the importance of using contextual information in biomedical text mining. Finally, they discuss the limitations of their method and suggest future directions for improving the ranking of indirect connections in literature-based discovery.
Throughout the article, the authors use simple language and engaging analogies to explain complex concepts. For instance, they describe MeSH terms as "building blocks" that can be combined to form a "vast biomedical dictionary." By demystifying these concepts, the authors make it easier for readers to understand the importance of contextual information in biomedical text mining.
In summary, the article highlights the significance of incorporating contextual information into biomedical text mining, particularly when linking concept associations. The proposed methodology offers a novel approach to ranking indirect connections between MeSH terms, which can improve the accuracy of link predictions. By leveraging this knowledge, researchers can enhance the efficiency and effectiveness of literature-based discovery in biomedicine.
Artificial Intelligence, Computer Science
Ranking Indirect Connections in Literature-Based Discovery: The Role of Medical Subject Headings
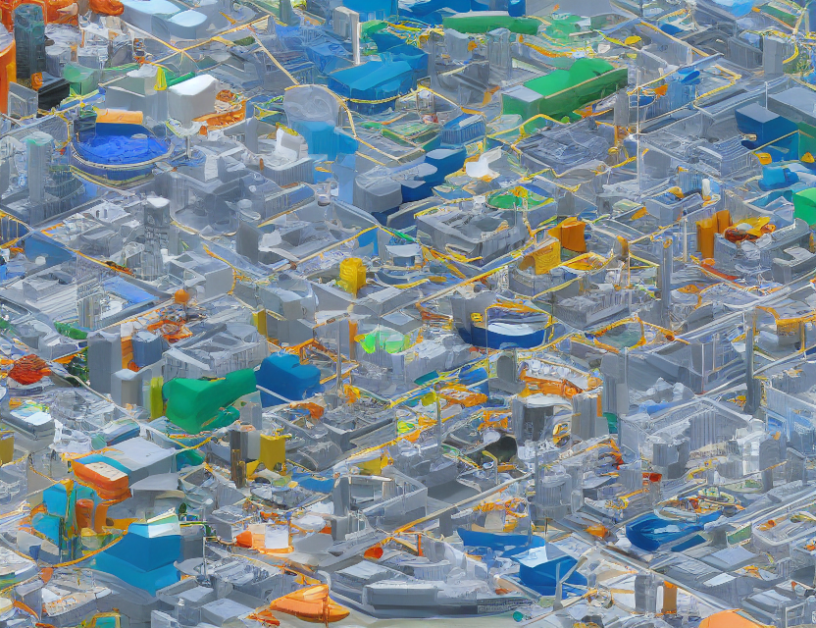