This research article discusses the development of a new dataset for image captioning, called "TextCaps," which is designed to improve the performance of automatic image captioning models by incorporating both visual and linguistic information. The dataset consists of over 10,000 images with corresponding captions, each carefully crafted to include both object recognition and language understanding components. The authors propose a novel approach to constructing the dataset, which involves identifying and removing any duplicate or redundant captions, and then manually correcting and augmenting the remaining captions to ensure they are accurate and informative.
The article highlights several key features of the TextCaps dataset, including its diverse range of objects, scenes, and styles, as well as its ability to capture complex contextual relationships between visual elements and language. The authors also demonstrate the effectiveness of their approach by showcasing how the dataset can be used to improve the performance of state-of-the-art image captioning models.
To create the TextCaps dataset, the authors first collected a large dataset of images from various sources, including online repositories and datasets. They then applied a series of filters to remove any duplicate or irrelevant images, resulting in a final dataset of over 10,000 unique images. Next, they carefully crafted each caption by identifying the object(s) depicted in each image and describing them in a clear and concise manner.
One innovative aspect of the TextCaps dataset is its focus on incorporating both visual and linguistic information. Unlike other datasets, which often rely solely on textual descriptions or visual features, TextCaps combines both to create a more comprehensive understanding of how language and vision interact in image captioning. This allows the models to learn not only how to recognize objects in an image but also how to use language to describe them effectively.
The authors also propose several novel evaluation metrics for assessing the performance of image captioning models on TextCaps, including a combination of automated and human-generated evaluations. These metrics allow researchers to evaluate their models not only against a baseline but also against a set of rigorously defined quality criteria, providing a more nuanced understanding of their performance.
Overall, the TextCaps dataset represents a significant advancement in the field of image captioning, providing a rich and diverse source of data for researchers to build upon and improve. Its ability to capture complex contextual relationships between visual elements and language makes it an ideal platform for developing more sophisticated and accurate image captioning models in the future.
Computer Science, Computer Vision and Pattern Recognition
Referitgame: Connecting Language and Vision with Crowdsourced Dense Image Annotations
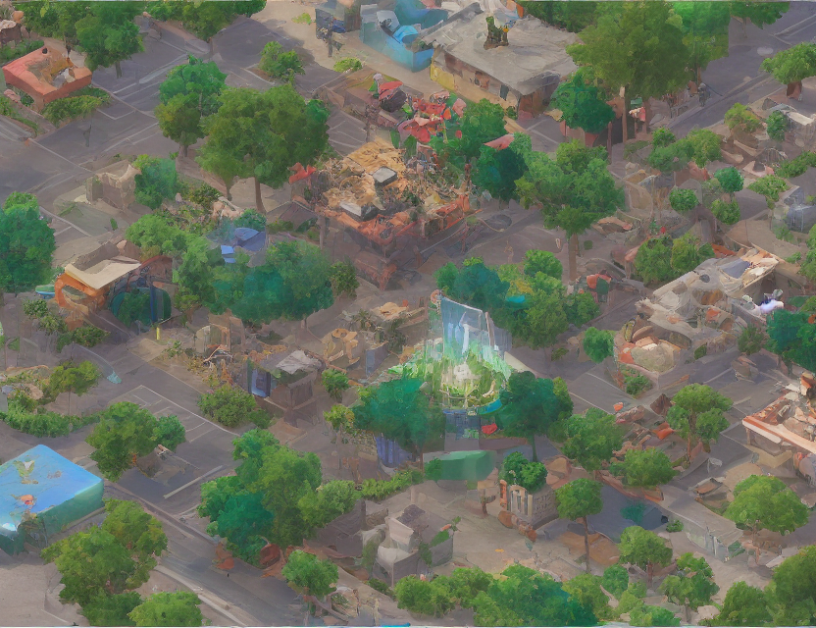