AutoML is a rapidly growing field that seeks to make machine learning (ML) more accessible and efficient for non-experts. By automating many of the time-consuming and resource-intensive tasks involved in ML, such as data preparation, feature engineering, hyperparameter tuning, and model selection, AutoML aims to democratize access to the power of ML.
To understand how AutoML works, let’s consider an analogy: imagine you want to bake a cake but don’t have any experience in the kitchen. You could try to follow a recipe step-by-step, but this can be time-consuming and overwhelming if you don’t know what you’re doing. AutoML is like having a magic cake-making machine that takes the recipe and does all the hard work for you, including measuring the ingredients, mixing them together, and baking the cake to perfection.
AutoML solutions can be divided into three main categories: supervised, unsupervised, and reinforcement learning. Each of these categories has its own strengths and weaknesses, and AutoML algorithms are designed to help users navigate these complex waters with ease. For example, linear regression is a well-established technique used in supervised learning to predict continuous outcomes based on input features. However, this process can be time-consuming and require a lot of expertise, especially when dealing with large datasets. AutoML algorithms like gradient boosting can simplify this process by automating the feature engineering and hyperparameter tuning tasks, allowing users to focus solely on the data analysis and model building.
However, there are challenges that must be addressed in order to fully realize the potential of AutoML. One major challenge is ensuring interpretability and transparency in AutoML solutions, particularly in highly regulated domains like the networking domain. This requires building trust and ethical considerations into the development process. Another challenge is addressing security concerns posed by adversarial attacks, which can compromise the accuracy and reliability of ML models.
Despite these challenges, the potential benefits of AutoML are significant, and it is an area that is likely to see continued growth and development in the years to come. By automating many of the time-consuming and resource-intensive tasks involved in ML, AutoML can greatly reduce the time and resources required to develop and deploy ML models, making ML more accessible to a broader audience without requiring extensive expertise in the field. This capability has the potential to revolutionize the way businesses operate, giving them the ability to leverage data-driven insights for decision-making and product development.
In conclusion, AutoML is a powerful tool that can greatly simplify and accelerate the ML process, making it more accessible to non-experts and democratizing access to the power of ML. While there are challenges that must be addressed, the potential benefits of AutoML make it an area worth watching in the coming years.
Computer Science, Networking and Internet Architecture
A Comprehensive Overview of AutoML: A Survey of Machine Learning and Articial Intelligence
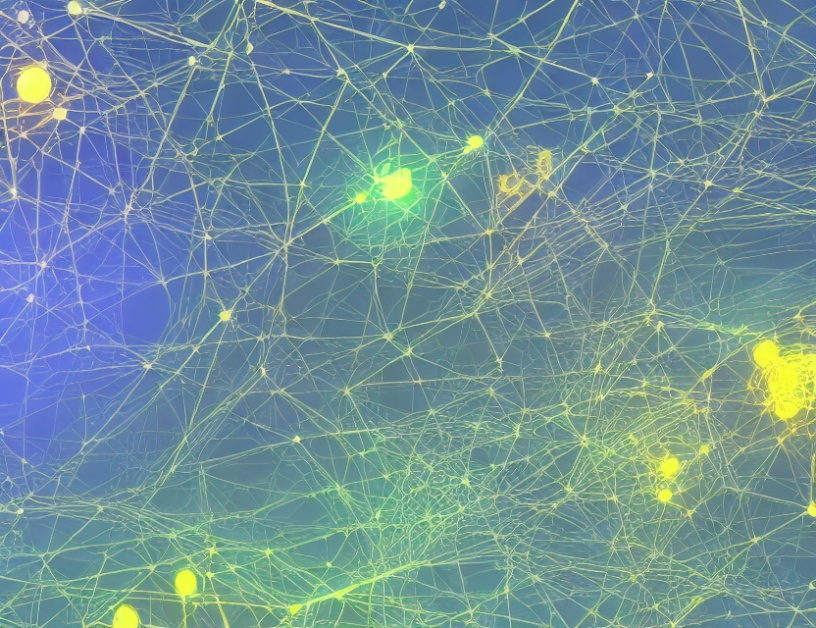