In this article, researchers propose a novel approach to assign molecules to spatial bins based on their properties and location in cells. The method is designed to improve the accuracy of cell segmentation and enable the analysis of cellular structures at a finer scale.
To begin with, the authors explain that traditional methods of assigning molecules to spatial bins are limited by their reliance on predefined rules or manual curation. These methods can be time-consuming and prone to errors, which can hinder the interpretation of results.
The proposed approach uses a deep learning model called graph convolutional neural network (GCNN) to learn abstract features from the spatial distribution of molecules in cells. The GCNN is trained on a large dataset of labeled cell images, allowing it to recognize patterns and relationships between molecules that are not easily visible by eye.
The authors then describe how the GCNN is used to compute attractive and repulsive edge strengths between molecules based on their spatial proximity. These edge strengths are used to construct a graph, where each molecule is represented as a node in the graph and edges connect molecules that are close to each other in space.
Next, the authors explain how the graph is partitioned into clusters of highly connected nodes, which correspond to different spatial bins. The size of these bins can vary depending on the specific application and experimental conditions. By analyzing the distribution of molecules within each bin, researchers can gain insights into the structure and function of cells at a finer scale than previously possible.
The authors also discuss several key advantages of their approach. Firstly, it allows for the automated segmentation of cells without the need for instance segmentation, which can be time-consuming and challenging. Secondly, it enables the analysis of cellular structures at multiple scales by adjusting the size of the spatial bins. Finally, it provides a more accurate representation of molecular distribution within cells than traditional methods by taking into account the spatial context of molecules.
In conclusion, the proposed approach offers a powerful tool for analyzing cellular structures at a finer scale than ever before. By leveraging deep learning and graph theory, researchers can automate the process of assigning molecules to spatial bins, enabling them to focus on more complex analyses that reveal the intricate workings of cells.
Computer Science, Information Theory
Adaptive Cell Segmentation for Spatial Transcriptomics Analysis
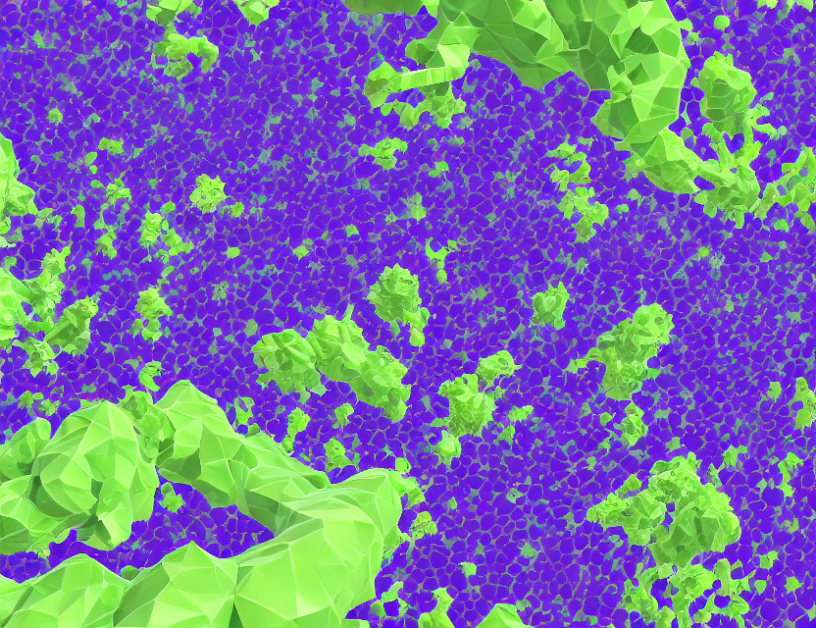