In this study, researchers aimed to create personalized coaching messages for weight loss based on participants’ own data trends. They used a combination of machine learning algorithms and human coding to generate these messages. The participants were asked to rate their agreement with each message, and the researchers evaluated which messages were most effective at encouraging healthy behaviors.
The study found that participants wanted coaching messages that were both concise and personalized. They also desired more detailed explanations within the messages regarding the data’s implications and a desire for the data to be referenced more consistently in supporting the advice given. Additionally, many participants noted that the messages served as valuable reminders of their behavioral goals and the skills they acquired during group treatment sessions, thereby aiding them in maintaining their progress.
The researchers used a theme-based approach to analyze the qualitative data, which involved identifying overarching themes from the codes created by participants. They collaboratively revised these themes and refined them by revisiting the data, ensuring each code matched a theme. The study had 41 participants with an average age of 53 years old and an average baseline BMI of 34.32.
The article provides valuable insights into how personalized coaching messages can be created using machine learning algorithms and human coding, and the importance of considering the full context of participants’ data trends to offer more targeted behavior suggestions. The study demonstrates that by combining machine learning with human input, it is possible to create personalized coaching messages that are both concise and effective in encouraging healthy behaviors.
In conclusion, this article highlights the potential of using machine learning algorithms in combination with human coding to create personalized coaching messages for weight loss. The study demonstrates that by considering the full context of participants’ data trends, these messages can be tailored to provide more targeted behavior suggestions, making them more effective in encouraging healthy behaviors.
Computation and Language, Computer Science
AI-Generated Messages in Weight Loss Treatment: A Qualitative Analysis
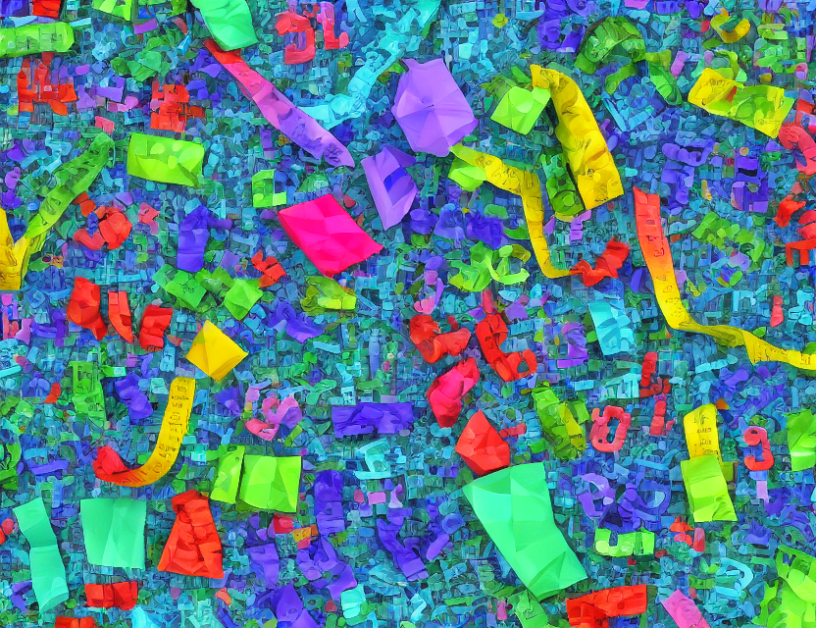