Conclusion: In conclusion, the article proposes a novel feature extractor called Structure-aware Feature Extractor (SAFE) to capture both semantic distance and structural similarity between entities in a question. SAFE outperforms baseline models in retrieving relevant examples for in-context learning tasks and demonstrates its applicability to various NLP tasks. The proposed feature extractor provides a useful contribution to the field of in-context learning by combining semantic distance and structural similarity for improving the performance of in-context learning models.
Computation and Language, Computer Science
Deduplication Task: Efficient Comparison and Removal of Duplicate Examples
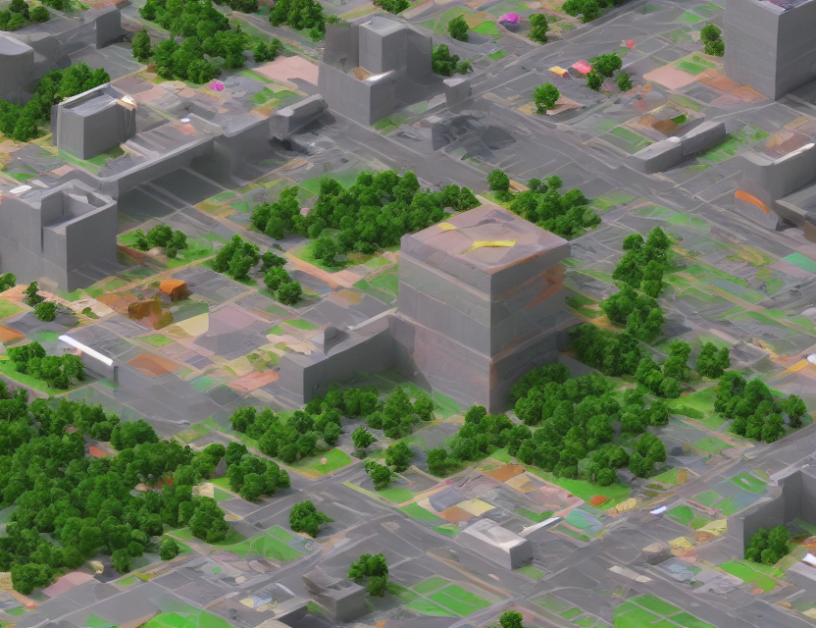